http://www.asyura2.com/14/it12/msg/295.html
Tweet |
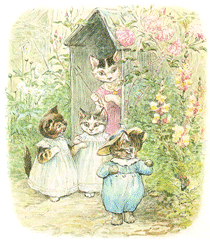
Hello quantum world! Google publishes landmark quantum supremacy claim
The company says that its quantum computer is the first to perform a calculation that would be practically impossible for a classical machine.
Elizabeth Gibney
Optical image of the Sycamore chip.
The Sycamore chip is composed of 54 qubits, each made of superconducting loops.Credit: Erik Lucero
Scientists at Google say that they have achieved quantum supremacy, a long-awaited milestone in quantum computing. The announcement, published in Nature on 23 October, follows a leak of an early version of the paper five weeks ago, which Google did not comment on at the time.
In a world first, a team led by John Martinis, an experimental physicist at the University of California, Santa Barbara, and Google in Mountain View, California, says that its quantum computer carried out a specific calculation that is beyond the practical capabilities of regular, ‘classical’ machines1. The same calculation would take even the best classical supercomputer 10,000 years to complete, Google estimates.
Quantum supremacy has long been seen as a milestone because it proves that quantum computers can outperform classical computers, says Martinis. Although the advantage has now been proved only for a very specific case, it shows physicists that quantum mechanics works as expected when harnessed in a complex problem.
“It looks like Google has given us the first experimental evidence that quantum speed-up is achievable in a real-world system,” says Michelle Simmons, a quantum physicist at the University of New South Wales in Sydney, Australia.
Martinis likens the experiment to a 'Hello World' programme, which tests a new system by instructing it to display that phrase; it's not especially useful in itself, but it tells Google that the quantum hardware and software are working correctly, he says.
The feat was first reported in September by the Financial Times and other outlets, after an early version of the paper was leaked on the website of NASA, which collaborates with Google on quantum computing, before being quickly taken down. At that time, the company did not confirm that it had written the paper, nor would it comment on the stories.
Although the calculation Google chose — checking the outputs from a quantum random-number generator — has limited practical applications, “the scientific achievement is huge, assuming it stands, and I’m guessing it will”, says Scott Aaronson, a theoretical computer scientist at the University of Texas at Austin.
Researchers outside Google are already trying to improve on the classical algorithms used to tackle the problem, in hopes of bringing down the firm's 10,000-year estimate. IBM, a rival to Google in building the world’s best quantum computers, reported in a preprint on 21 October that the problem could be solved in just 2.5 days using a different classical technique2. That paper has not been peer reviewed. If IBM is correct, it would reduce Google’s feat to demonstrating a quantum ‘advantage’ — doing a calculation much faster than a classical computer, but not something that is beyond its reach. This would still be a significant landmark, says Simmons. “As far as I’m aware, that’s the first time that’s been demonstrated, so that’s definitely a big result.”
Quick solutions
Quantum computers work in a fundamentally different way from classical machines: a classical bit is either a 1 or a 0, but a quantum bit, or qubit, can exist in multiple states at once. When qubits are inextricably linked, physicists can, in theory, exploit the interference between their wave-like quantum states to perform calculations that might otherwise take millions of years.
Physicists think that quantum computers might one day run revolutionary algorithms that could, for example, search unwieldy databases or factor large numbers — including, importantly, those used in encryption. But those applications are still decades away. The more qubits are linked, the harder it is to maintain their fragile states while the device is operating. Google’s algorithm runs on a quantum chip composed of 54 qubits, each made of superconducting loops. But this is a tiny fraction of the one million qubits that could be needed for a general-purpose machine.
The task Google set for its quantum computer is “a bit of a weird one”, says Christopher Monroe, a physicist at the University of Maryland in College Park. Google physicists first crafted the problem in 2016, and it was designed to be extremely difficult for an ordinary computer to solve. The team challenged its computer, known as Sycamore, to describe the likelihood of different outcomes from a quantum version of a random-number generator. They do this by running a circuit that passes 53 qubits through a series of random operations. This generates a 53-digit string of 1s and 0s — with a total of 253 possible combinations (only 53 qubits were used because one of Sycamore’s 54 was broken). The process is so complex that the outcome is impossible to calculate from first principles, and is therefore effectively random. But owing to interference between qubits, some strings of numbers are more likely to occur than others. This is similar to rolling a loaded die — it still produces a random number, even though some outcomes are more likely than others.
Sycamore calculated the probability distribution by sampling the circuit — running it one million times and measuring the observed output strings. The method is similar to rolling the die to reveal its bias. In one sense, says Monroe, the machine is doing something scientists do every day: using an experiment to find the answer to a quantum problem that is impossible to calculate classically. The key difference, he says, is that Google’s computer is not single-purpose, but programmable, and could be applied to a quantum circuit with any settings.
Memner of the Google quantum team work on a cryostat
Google's quantum computer excels at checking the outputs of a quantum random number generator.Credit: Erik Lucero
Verifying the solution was a further challenge. To do that, the team compared the results with those from simulations of smaller and simpler versions of the circuits, which were done by classical computers — including the Summit supercomputer at Oak Ridge National Laboratory in Tennessee. Extrapolating from these examples, the Google team estimates that simulating the full circuit would take 10,000 years even on a computer with one million processing units (equivalent to around 100,000 desktop computers). Sycamore took just 3 minutes and 20 seconds.
Google thinks their evidence for quantum supremacy is airtight. Even if external researchers cut the time it takes to do the classical simulation, quantum hardware is improving — meaning that for this problem, conventional computers are unlikely to ever catch up, says Hartmut Neven, who runs Google’s quantum-computing team.
Limited applications
Monroe says that Google’s achievement might benefit quantum computing by attracting more computer scientists and engineers to the field. But he also warns that the news could create the impression that quantum computers are closer to mainstream practical applications than they really are. “The story on the street is ‘they’ve finally beaten a regular computer: so here we go, two years and we’ll have one in our house’,” he says.
In reality, Monroe adds, scientists are yet to show that a programmable quantum computer can solve a useful task that cannot be done any other way, such as by calculating the electronic structure of a particular molecule — a fiendish problem that requires modelling multiple quantum interactions. Another important step, says Aaronson, is demonstrating quantum supremacy in an algorithm that uses a process known as error correction — a method to correct for noise-induced errors that would otherwise ruin a calculation. Physicists think this will be essential to getting quantum computers to function at scale.
Google is working towards both of these milestones, says Martinis, and will reveal the results of its experiments in the coming months.
Aaronson says that the experiment Google devised to demonstrate quantum supremacy might have practical applications: he has created a protocol to use such a calculation to prove to a user that the bits generated by a quantum random-number generator really are random. This could be useful, for example, in cryptography and some cryptocurrencies, whose security relies on random keys.
Google engineers had to carry out a raft of improvements to their hardware to run the algorithm, including building new electronics to control the quantum circuit and devising a new way to connect qubits, says Martinis. “This is really the basis of how we’re going to scale up in the future. We think this basic architecture is the way forward,” he says.
Nature 574, 461-462 (2019)
doi: 10.1038/d41586-019-03213-z
References
1.
Arute, F. et al. Nature 574, 505–510 (2019).
ArticleGoogle Scholar
2.
Pednault, E. et al. Preprint at https://arxiv.org/abs/1910.09534 (2019).
Download references
show less
Latest on:
Computer science
Quantum physics
Quantum computing takes flight
Quantum computing takes flight
NEWS & VIEWS 23 OCT 19
The social-media war, reclaiming classics from the alt-right, and a fusion of physics and dance: New in paperback
The social-media war, reclaiming classics from the alt-right, and a fusion of physics and dance: New in paperback
BOOKS & ARTS 15 OCT 19
Why deep-learning AIs are so easy to fool
Why deep-learning AIs are so easy to fool
NEWS FEATURE
https://www.nature.com/articles/d41586-019-03213-z
Quantum computing takes flight
A programmable quantum computer has been reported to outperform the most powerful conventional computers in a specific task — a milestone in computing comparable in importance to the Wright brothers’ first flights.
William D. Oliver
Quantum computers promise to perform certain tasks much faster than ordinary (classical) computers. In essence, a quantum computer carefully orchestrates quantum effects (superposition, entanglement and interference) to explore a huge computational space and ultimately converge on a solution, or solutions, to a problem. If the numbers of quantum bits (qubits) and operations reach even modest levels, carrying out the same task on a state-of-the-art supercomputer becomes intractable on any reasonable timescale — a regime termed quantum computational supremacy1. However, reaching this regime requires a robust quantum processor, because each additional imperfect operation incessantly chips away at overall performance. It has therefore been questioned whether a sufficiently large quantum computer could ever be controlled in practice. But now, in a paper in Nature, Arute et al.2 report quantum supremacy using a 53-qubit processor.
Read the paper: Quantum supremacy using a programmable superconducting processor
Arute and colleagues chose a task that is related to random-number generation: namely, sampling the output of a pseudo-random quantum circuit. This task is implemented by a sequence of operational cycles, each of which applies operations called gates to every qubit in an n-qubit processor. These operations include randomly selected single-qubit gates and prescribed two-qubit gates. The output is then determined by measuring each qubit.
The resulting strings of 0s and 1s are not uniformly distributed over all 2n possibilities. Instead, they have a preferential, circuit-dependent structure — with certain strings being much more likely than others because of quantum entanglement and quantum interference. Repeating the experiment and sampling a sufficiently large number of these solutions results in a distribution of likely outcomes. Simulating this probability distribution on a classical computer using even today’s leading algorithms becomes exponentially more challenging as the number of qubits and operational cycles is increased.
In their experiment, Arute et al. used a quantum processor dubbed Sycamore. This processor comprises 53 individually controllable qubits, 86 couplers (links between qubits) that are used to turn nearest-neighbour two-qubit interactions on or off, and a scheme to measure all of the qubits simultaneously. In addition, the authors used 277 digital-to-analog converter devices to control the processor.
When all the qubits were operated simultaneously, each single-qubit and two-qubit gate had approximately 99–99.9% fidelity — a measure of how similar an actual outcome of an operation is to the ideal outcome. The attainment of such fidelities is one of the remarkable technical achievements that enabled this work. Arute and colleagues determined the fidelities using a protocol known as cross-entropy benchmarking (XEB). This protocol was introduced last year3 and offers certain advantages over other methods for diagnosing systematic and random errors.
Promising ways to encode and manipulate quantum information
The authors’ demonstration of quantum supremacy involved sampling the solutions from a pseudo-random circuit implemented on Sycamore and then comparing these results to simulations performed on several powerful classical computers, including the Summit supercomputer at Oak Ridge National Laboratory in Tennessee (see go.nature.com/35zfbuu). Summit is currently the world’s leading supercomputer, capable of carrying out about 200 million billion operations per second. It comprises roughly 40,000 processor units, each of which contains billions of transistors (electronic switches), and has 250 million gigabytes of storage. Approximately 99% of Summit’s resources were used to perform the classical sampling.
Verifying quantum supremacy for the sampling problem is challenging, because this is precisely the regime in which classical simulations are infeasible. To address this issue, Arute et al. first carried out experiments in a classically verifiable regime using three different circuits: the full circuit, the patch circuit and the elided circuit (Fig. 1). The full circuit used all n qubits and was the hardest to simulate. The patch circuit cut the full circuit into two patches that each had about n/2 qubits and were individually much easier to simulate. Finally, the elided circuit made limited two-qubit connections between the two patches, resulting in a level of computational difficulty that is intermediate between those of the full circuit and the patch circuit.
Figure 1 | Three types of quantum circuit. Arute et al.2 demonstrate that a quantum processor containing 53 quantum bits (qubits) and 86 couplers (links between qubits) can complete a specific task much faster than an ordinary computer can simulate the same task. Their demonstration is based on three quantum circuits: the full circuit, the patch circuit and the elided circuit. The full circuit comprises all 53 qubits and is the hardest to simulate on an ordinary computer. The patch circuit cuts the full circuit into two patches that are each relatively easy to simulate. Finally, the elided circuit links these two patches using a reduced number of two-qubit operations along reintroduced two-qubit connections and is intermediate between the full and patch circuits, in terms of its ease of simulation.
The authors selected a simplified set of two-qubit gates and a limited number of cycles (14) to produce full, patch and elided circuits that could be simulated in a reasonable amount of time. Crucially, the classical simulations for all three circuits yielded consistent XEB fidelities for up to n = 53 qubits, providing evidence that the patch and elided circuits serve as good proxies for the full circuit. The simulations of the full circuit also matched calculations that were based solely on the individual fidelities of the single-qubit and two-qubit gates. This finding indicates that errors remain well described by a simple, localized model, even as the number of qubits and operations increases.
Arute and colleagues’ longest, directly verifiable measurement was performed on the full circuit (containing 53 qubits) over 14 cycles. The quantum processor took one million samples in 200 seconds to reach an XEB fidelity of 0.8% (with a sensitivity limit of roughly 0.1% owing to the sampling statistics). By comparison, performing the sampling task at 0.8% fidelity on a classical computer (containing about one million processor cores) took 130 seconds, and a precise classical verification (100% fidelity) took 5 hours. Given the immense disparity in physical resources, these results already show a clear advantage of quantum hardware over its classical counterpart.
The authors then extended the circuits into the not-directly-verifiable supremacy regime. They used a broader set of two-qubit gates to spread entanglement more widely across the full 53-qubit processor and increased the number of cycles from 14 to 20. The full circuit could not be simulated or directly verified in a reasonable amount of time, so Arute et al. simply archived these quantum data for future reference — in case extremely efficient classical algorithms are one day discovered that would enable verification. However, the patch-circuit, elided-circuit and calculated XEB fidelities all remained in agreement. When 53 qubits were operating over 20 cycles, the XEB fidelity calculated using these proxies remained greater than 0.1%. Sycamore sampled the solutions in a mere 200 seconds, whereas classical sampling at 0.1% fidelity would take 10,000 years, and full verification would take several million years.
This demonstration of quantum supremacy over today’s leading classical algorithms on the world’s fastest supercomputers is truly a remarkable achievement and a milestone for quantum computing. It experimentally suggests that quantum computers represent a model of computing that is fundamentally different from that of classical computers4. It also further combats criticisms5,6 about the controllability and viability of quantum computation in an extraordinarily large computational space (containing at least the 253 states used here).
However, much work is needed before quantum computers become a practical reality. In particular, algorithms will have to be developed that can be commercialized and operate on the noisy (error-prone) intermediate-scale quantum processors that will be available in the near term1. And researchers will need to demonstrate robust protocols for quantum error correction that will enable sustained, fault-tolerant operation in the longer term.
Arute and colleagues’ demonstration is in many ways reminiscent of the Wright brothers’ first flights. Their aeroplane, the Wright Flyer, wasn’t the first airborne vehicle to fly, and it didn’t solve any pressing transport problem. Nor did it herald the widespread adoption of planes or mark the beginning of the end for other modes of transport. Instead, the event is remembered for having shown a new operational regime — the self-propelled flight of an aircraft that was heavier than air. It is what the event represented, rather than what it practically accomplished, that was paramount. And so it is with this first report of quantum computational supremacy.
Nature 574, 487-488 (2019)
doi: 10.1038/d41586-019-03173-4
References
1.
Preskill, J. Preprint at https://arxiv.org/abs/1203.5813 (2012).
2.
Arute, F. et al. Nature 574, 505–511 (2019).
ArticleGoogle Scholar
3.
Boixo, S. et al. Nature Phys. 14, 595–600 (2018).
ArticleGoogle Scholar
4.
Bernstein, E. & Vazirani, U. Proc. 25th Annu. Symp. Theory Comput. (ACM, 1993).
5.
Dyakonov, M. The case against quantum computing. IEEE Spectrum (2018).
6.
Kalai, G. Preprint at https://arxiv.org/abs/1908.02499 (2019).
Download references
show less
Latest on:
Computer science
Quantum information
Quantum physics
Hello quantum world! Google publishes landmark quantum supremacy claim
Hello quantum world! Google publishes landmark quantum supremacy claim
NEWS 23 OCT 19
The social-media war, reclaiming classics from the alt-right, and a fusion of physics and dance: New in paperback
The social-media war, reclaiming classics from the alt-right, and a fusion of physics and dance: New in paperback
BOOKS & ARTS 15 OCT 19
Why deep-learning AIs are so easy to fool
Why deep-learning AIs are so easy to fool
NEWS FEATURE 09 OCT 19
https://www.nature.com/articles/d41586-019-03013-5
Quantum computing takes flight
A programmable quantum computer has been reported to outperform the most powerful conventional computers in a specific task — a milestone in computing comparable in importance to the Wright brothers’ first flights.
William D. Oliver
•
•
•
Quantum computers promise to perform certain tasks much faster than ordinary (classical) computers. In essence, a quantum computer carefully orchestrates quantum effects (superposition, entanglement and interference) to explore a huge computational space and ultimately converge on a solution, or solutions, to a problem. If the numbers of quantum bits (qubits) and operations reach even modest levels, carrying out the same task on a state-of-the-art supercomputer becomes intractable on any reasonable timescale — a regime termed quantum computational supremacy1. However, reaching this regime requires a robust quantum processor, because each additional imperfect operation incessantly chips away at overall performance. It has therefore been questioned whether a sufficiently large quantum computer could ever be controlled in practice. But now, in a paper in Nature, Arute et al.2 report quantum supremacy using a 53-qubit processor.
Read the paper: Quantum supremacy using a programmable superconducting processor
Arute and colleagues chose a task that is related to random-number generation: namely, sampling the output of a pseudo-random quantum circuit. This task is implemented by a sequence of operational cycles, each of which applies operations called gates to every qubit in an n-qubit processor. These operations include randomly selected single-qubit gates and prescribed two-qubit gates. The output is then determined by measuring each qubit.
The resulting strings of 0s and 1s are not uniformly distributed over all 2n possibilities. Instead, they have a preferential, circuit-dependent structure — with certain strings being much more likely than others because of quantum entanglement and quantum interference. Repeating the experiment and sampling a sufficiently large number of these solutions results in a distribution of likely outcomes. Simulating this probability distribution on a classical computer using even today’s leading algorithms becomes exponentially more challenging as the number of qubits and operational cycles is increased.
In their experiment, Arute et al. used a quantum processor dubbed Sycamore. This processor comprises 53 individually controllable qubits, 86 couplers (links between qubits) that are used to turn nearest-neighbour two-qubit interactions on or off, and a scheme to measure all of the qubits simultaneously. In addition, the authors used 277 digital-to-analog converter devices to control the processor.
When all the qubits were operated simultaneously, each single-qubit and two-qubit gate had approximately 99–99.9% fidelity — a measure of how similar an actual outcome of an operation is to the ideal outcome. The attainment of such fidelities is one of the remarkable technical achievements that enabled this work. Arute and colleagues determined the fidelities using a protocol known as cross-entropy benchmarking (XEB). This protocol was introduced last year3 and offers certain advantages over other methods for diagnosing systematic and random errors.
Promising ways to encode and manipulate quantum information
The authors’ demonstration of quantum supremacy involved sampling the solutions from a pseudo-random circuit implemented on Sycamore and then comparing these results to simulations performed on several powerful classical computers, including the Summit supercomputer at Oak Ridge National Laboratory in Tennessee (see go.nature.com/35zfbuu). Summit is currently the world’s leading supercomputer, capable of carrying out about 200 million billion operations per second. It comprises roughly 40,000 processor units, each of which contains billions of transistors (electronic switches), and has 250 million gigabytes of storage. Approximately 99% of Summit’s resources were used to perform the classical sampling.
Verifying quantum supremacy for the sampling problem is challenging, because this is precisely the regime in which classical simulations are infeasible. To address this issue, Arute et al. first carried out experiments in a classically verifiable regime using three different circuits: the full circuit, the patch circuit and the elided circuit (Fig. 1). The full circuit used all n qubits and was the hardest to simulate. The patch circuit cut the full circuit into two patches that each had about n/2 qubits and were individually much easier to simulate. Finally, the elided circuit made limited two-qubit connections between the two patches, resulting in a level of computational difficulty that is intermediate between those of the full circuit and the patch circuit.
Figure 1 | Three types of quantum circuit. Arute et al.2 demonstrate that a quantum processor containing 53 quantum bits (qubits) and 86 couplers (links between qubits) can complete a specific task much faster than an ordinary computer can simulate the same task. Their demonstration is based on three quantum circuits: the full circuit, the patch circuit and the elided circuit. The full circuit comprises all 53 qubits and is the hardest to simulate on an ordinary computer. The patch circuit cuts the full circuit into two patches that are each relatively easy to simulate. Finally, the elided circuit links these two patches using a reduced number of two-qubit operations along reintroduced two-qubit connections and is intermediate between the full and patch circuits, in terms of its ease of simulation.
The authors selected a simplified set of two-qubit gates and a limited number of cycles (14) to produce full, patch and elided circuits that could be simulated in a reasonable amount of time. Crucially, the classical simulations for all three circuits yielded consistent XEB fidelities for up to n = 53 qubits, providing evidence that the patch and elided circuits serve as good proxies for the full circuit. The simulations of the full circuit also matched calculations that were based solely on the individual fidelities of the single-qubit and two-qubit gates. This finding indicates that errors remain well described by a simple, localized model, even as the number of qubits and operations increases.
Arute and colleagues’ longest, directly verifiable measurement was performed on the full circuit (containing 53 qubits) over 14 cycles. The quantum processor took one million samples in 200 seconds to reach an XEB fidelity of 0.8% (with a sensitivity limit of roughly 0.1% owing to the sampling statistics). By comparison, performing the sampling task at 0.8% fidelity on a classical computer (containing about one million processor cores) took 130 seconds, and a precise classical verification (100% fidelity) took 5 hours. Given the immense disparity in physical resources, these results already show a clear advantage of quantum hardware over its classical counterpart.
The authors then extended the circuits into the not-directly-verifiable supremacy regime. They used a broader set of two-qubit gates to spread entanglement more widely across the full 53-qubit processor and increased the number of cycles from 14 to 20. The full circuit could not be simulated or directly verified in a reasonable amount of time, so Arute et al. simply archived these quantum data for future reference — in case extremely efficient classical algorithms are one day discovered that would enable verification. However, the patch-circuit, elided-circuit and calculated XEB fidelities all remained in agreement. When 53 qubits were operating over 20 cycles, the XEB fidelity calculated using these proxies remained greater than 0.1%. Sycamore sampled the solutions in a mere 200 seconds, whereas classical sampling at 0.1% fidelity would take 10,000 years, and full verification would take several million years.
This demonstration of quantum supremacy over today’s leading classical algorithms on the world’s fastest supercomputers is truly a remarkable achievement and a milestone for quantum computing. It experimentally suggests that quantum computers represent a model of computing that is fundamentally different from that of classical computers4. It also further combats criticisms5,6 about the controllability and viability of quantum computation in an extraordinarily large computational space (containing at least the 253 states used here).
However, much work is needed before quantum computers become a practical reality. In particular, algorithms will have to be developed that can be commercialized and operate on the noisy (error-prone) intermediate-scale quantum processors that will be available in the near term1. And researchers will need to demonstrate robust protocols for quantum error correction that will enable sustained, fault-tolerant operation in the longer term.
Arute and colleagues’ demonstration is in many ways reminiscent of the Wright brothers’ first flights. Their aeroplane, the Wright Flyer, wasn’t the first airborne vehicle to fly, and it didn’t solve any pressing transport problem. Nor did it herald the widespread adoption of planes or mark the beginning of the end for other modes of transport. Instead, the event is remembered for having shown a new operational regime — the self-propelled flight of an aircraft that was heavier than air. It is what the event represented, rather than what it practically accomplished, that was paramount. And so it is with this first report of quantum computational supremacy.
Nature 574, 487-488 (2019)
doi: 10.1038/d41586-019-03173-4
References
1. 1.
Preskill, J. Preprint at https://arxiv.org/abs/1203.5813 (2012).
o
2. 2.
Arute, F. et al. Nature 574, 505–511 (2019).
o
Article
Google Scholar
3. 3.
Boixo, S. et al. Nature Phys. 14, 595–600 (2018).
o
Article
Google Scholar
4. 4.
Bernstein, E. & Vazirani, U. Proc. 25th Annu. Symp. Theory Comput. (ACM, 1993).
o
5. 5.
Dyakonov, M. The case against quantum computing. IEEE Spectrum (2018).
o
6. 6.
Kalai, G. Preprint at https://arxiv.org/abs/1908.02499 (2019).
o
Download references
show less
Latest on:
Computer science
Quantum information
Quantum physics
Hello quantum world! Google publishes landmark quantum supremacy claim
NEWS 23 OCT 19
The social-media war, reclaiming classics from the alt-right, and a fusion of physics and dance: New in paperback
BOOKS & ARTS 15 OCT 19
Why deep-learning AIs are so easy to fool
NEWS FEATURE 09 OCT 19
https://www.nature.com/articles/d41586-019-03173-4
Why deep-learning AIs are so easy to fool
Artificial-intelligence researchers are trying to fix the flaws of neural networks.
Douglas Heaven
Illustration by Edgar Bąk
PDF version
A self-driving car approaches a stop sign, but instead of slowing down, it accelerates into the busy intersection. An accident report later reveals that four small rectangles had been stuck to the face of the sign. These fooled the car’s onboard artificial intelligence (AI) into misreading the word ‘stop’ as ‘speed limit 45’.
Such an event hasn’t actually happened, but the potential for sabotaging AI is very real. Researchers have already demonstrated how to fool an AI system into misreading a stop sign, by carefully positioning stickers on it1. They have deceived facial-recognition systems by sticking a printed pattern on glasses or hats. And they have tricked speech-recognition systems into hearing phantom phrases by inserting patterns of white noise in the audio.
These are just some examples of how easy it is to break the leading pattern-recognition technology in AI, known as deep neural networks (DNNs). These have proved incredibly successful at correctly classifying all kinds of input, including images, speech and data on consumer preferences. They are part of daily life, running everything from automated telephone systems to user recommendations on the streaming service Netflix. Yet making alterations to inputs — in the form of tiny changes that are typically imperceptible to humans — can flummox the best neural networks around.
These problems are more concerning than idiosyncratic quirks in a not-quite-perfect technology, says Dan Hendrycks, a PhD student in computer science at the University of California, Berkeley. Like many scientists, he has come to see them as the most striking illustration that DNNs are fundamentally brittle: brilliant at what they do until, taken into unfamiliar territory, they break in unpredictable ways.
Sources: Stop sign: Ref. 1; Penguin: Ref. 5
That could lead to substantial problems. Deep-learning systems are increasingly moving out of the lab into the real world, from piloting self-driving cars to mapping crime and diagnosing disease. But pixels maliciously added to medical scans could fool a DNN into wrongly detecting cancer, one study reported this year2. Another suggested that a hacker could use these weaknesses to hijack an online AI-based system so that it runs the invader’s own algorithms3.
In their efforts to work out what’s going wrong, researchers have discovered a lot about why DNNs fail. “There are no fixes for the fundamental brittleness of deep neural networks,” argues François Chollet, an AI engineer at Google in Mountain View, California. To move beyond the flaws, he and others say, researchers need to augment pattern-matching DNNs with extra abilities: for instance, making AIs that can explore the world for themselves, write their own code and retain memories. These kinds of system will, some experts think, form the story of the coming decade in AI research.
Reality check
In 2011, Google revealed a system that could recognize cats in YouTube videos, and soon after came a wave of DNN-based classification systems. “Everybody was saying, ‘Wow, this is amazing, computers are finally able to understand the world,’” says Jeff Clune at the University of Wyoming in Laramie, who is also a senior research manager at Uber AI Labs in San Francisco, California.
But AI researchers knew that DNNs do not actually understand the world. Loosely modelled on the architecture of the brain, they are software structures made up of large numbers of digital neurons arranged in many layers. Each neuron is connected to others in layers above and below it.
The idea is that features of the raw input coming into the bottom layers — such as pixels in an image — trigger some of those neurons, which then pass on a signal to neurons in the layer above according to simple mathematical rules. Training a DNN network involves exposing it to a massive collection of examples, each time tweaking the way in which the neurons are connected so that, eventually, the top layer gives the desired answer — such as always interpreting a picture of a lion as a lion, even if the DNN hasn’t seen that picture before.
A first big reality check came in 2013, when Google researcher Christian Szegedy and his colleagues posted a preprint called ‘Intriguing properties of neural networks’4. The team showed that it was possible to take an image — of a lion, for example — that a DNN could identify and, by altering a few pixels, convince the machine that it was looking at something different, such as a library. The team called the doctored images ‘adversarial examples’.
A year later, Clune and his then-PhD student Anh Nguyen, together with Jason Yosinski at Cornell University in Ithaca, New York, showed that it was possible to make DNNs see things that were not there, such as a penguin in a pattern of wavy lines5. “Anybody who has played with machine learning knows these systems make stupid mistakes once in a while,” says Yoshua Bengio at the University of Montreal in Canada, who is a pioneer of deep learning. “What was a surprise was the type of mistake,” he says. “That was pretty striking. It’s a type of mistake we would not have imagined would happen.”
New types of mistake have come thick and fast. Last year, Nguyen, who is now at Auburn University in Alabama, showed that simply rotating objects in an image was sufficient to throw off some of the best image classifiers around6. This year, Hendrycks and his colleagues reported that even unadulterated, natural images can still trick state-of-the-art classifiers into making unpredictable gaffes, such as identifying a mushroom as a pretzel or a dragonfly as a manhole cover7.
The issue goes beyond object recognition: any AI that uses DNNs to classify inputs — such as speech — can be fooled. AIs that play games can be sabotaged: in 2017, computer scientist Sandy Huang, a PhD student at the University of California, Berkeley, and her colleagues focused on DNNs that had been trained to beat Atari video games through a process called reinforcement learning8. In this approach, an AI is given a goal and, in response to a range of inputs, learns through trial and error what to do to reach that goal. It is the technology behind superhuman game-playing AIs such as AlphaZero and the poker bot Pluribus. Even so, Huang’s team was able to make their AIs lose games by adding one or two random pixels to the screen.
Earlier this year, AI PhD student Adam Gleave at the University of California, Berkeley, and his colleagues demonstrated that it is possible to introduce an agent to an AI’s environment that acts out an ‘adversarial policy’ designed to confuse the AI’s responses9. For example, an AI footballer trained to kick a ball past an AI goalkeeper in a simulated environment loses its ability to score when the goalkeeper starts to behave in unexpected ways, such as collapsing on the ground.
A simulated soccer penalty shootout between two Humanoid robots displayed with and without a adversarial policy
An AI footballer in a simulated penalty-shootout is confused when the AI goalkeeper enacts an ‘adversarial policy’: falling to the floor (right).Credit: Adam Gleave/Ref. 9
Knowing where a DNN’s weak spots are could even let a hacker take over a powerful AI. One example of that came last year, when a team from Google showed that it was possible to use adversarial examples not only to force a DNN to make specific mistakes, but also to reprogram it entirely — effectively repurposing an AI trained on one task to do another3.
Many neural networks, such as those that learn to understand language, can, in principle, be used to encode any other computer program. “In theory, you can turn a chatbot into whatever programme you want,” says Clune. “This is where the mind starts to boggle.” He imagines a situation in the near future in which hackers could hijack neural nets in the cloud to run their own spambot-dodging algorithms.
For computer scientist Dawn Song at the University of California, Berkeley, DNNs are like sitting ducks. “There are so many different ways that you can attack a system,” she says. “And defence is very, very difficult.”
With great power comes great fragility
DNNs are powerful because their many layers mean they can pick up on patterns in many different features of an input when attempting to classify it. An AI trained to recognize aircraft might find that features such as patches of colour, texture or background are just as strong predictors as the things that we would consider salient, such as wings. But this also means that a very small change in the input can tip it over into what the AI considers an apparently different state.
One answer is simply to throw more data at the AI; in particular, to repeatedly expose the AI to problematic cases and correct its errors. In this form of ‘adversarial training’, as one network learns to identify objects, a second tries to change the first network’s inputs so that it makes mistakes. In this way, adversarial examples become part of a DNN’s training data.
Hendrycks and his colleagues have suggested quantifying a DNN’s robustness against making errors by testing how it performs against a large range of adversarial examples. However, training a network to withstand one kind of attack could weaken it against others, they say. And researchers led by Pushmeet Kohli at Google DeepMind in London are trying to inoculate DNNs against making mistakes. Many adversarial attacks work by making tiny tweaks to the component parts of an input — such as subtly altering the colour of pixels in an image — until this tips a DNN over into a misclassification. Kohli’s team has suggested that a robust DNN should not change its output as a result of small changes in its input, and that this property might be mathematically incorporated into the network, constraining how it learns.
For the moment, however, no one has a fix on the overall problem of brittle AIs. The root of the issue, says Bengio, is that DNNs don’t have a good model of how to pick out what matters. When an AI sees a doctored image of a lion as a library, a person still sees a lion because they have a mental model of the animal that rests on a set of high-level features — ears, a tail, a mane and so on — that lets them abstract away from low-level arbitrary or incidental details. “We know from prior experience which features are the salient ones,” says Bengio. “And that comes from a deep understanding of the structure of the world.”
One attempt to address this is to combine DNNs with symbolic AI, which was the dominant paradigm in AI before machine learning. With symbolic AI, machines reasoned using hard-coded rules about how the world worked, such as that it contains discrete objects and that they are related to one another in various ways. Some researchers, such as psychologist Gary Marcus at New York University, say hybrid AI models are the way forward. “Deep learning is so useful in the short term that people have lost sight of the long term,” says Marcus, who is a long-time critic of the current deep-learning approach. In May, he co-founded a start-up called Robust AI in Palo Alto, California, which aims to mix deep learning with rule-based AI techniques to develop robots that can operate safely alongside people. Exactly what the company is working on remains under wraps.
Even if rules can be embedded into DNNs, they are still only as good as the data they learn from. Bengio says that AI agents need to learn in richer environments that they can explore. For example, most computer-vision systems fail to recognize that a can of beer is cylindrical because they were trained on data sets of 2D images. That is why Nguyen and colleagues found it so easy to fool DNNs by presenting familiar objects from different perspectives. Learning in a 3D environment — real or simulated — will help.
But the way AIs do their learning also needs to change. “Learning about causality needs to be done by agents that do things in the world, that can experiment and explore,” says Bengio. Another deep-learning pioneer, Jürgen Schmidhuber at the Dalle Molle Institute for Artificial Intelligence Research in Manno, Switzerland, thinks along similar lines. Pattern recognition is extremely powerful, he says — good enough to have made companies such as Alibaba, Tencent, Amazon, Facebook and Google the most valuable in the world. “But there’s a much bigger wave coming,” he says. “And this will be about machines that manipulate the world and create their own data through their own actions.”
In a sense, AIs that use reinforcement learning to beat computer games are doing this already in artificial environments: by trial and error, they manipulate pixels on screen in allowed ways until they reach a goal. But real environments are much richer than the simulated or curated data sets on which most DNNs train today.
Robots that improvise
In a laboratory at the University of California, Berkeley, a robot arm rummages through clutter. It picks up a red bowl and uses it to nudge a blue oven glove a couple of centimetres to the right. It drops the bowl and picks up an empty plastic spray bottle. Then it explores the heft and shape of a paperback book. Over several days of non-stop sifting, the robot starts to get a feel for these alien objects and what it can do with them.
The robot arm is using deep learning to teach itself to use tools. Given a tray of objects, it picks up and looks at each in turn, seeing what happens when it moves them around and knocks one object into another.
Collection of clips of robots improvising with tools
Robots use deep learning to explore how to use 3D tools.Credit: Annie Xie
When researchers give the robot a goal — for instance, presenting it with an image of a nearly empty tray and specifying that the robot arrange objects to match that state — it improvises, and can work with objects it has not seen before, such as using a sponge to wipe objects off a table. It also figured out that clearing up using a plastic water bottle to knock objects out of the way is quicker than picking up those objects directly. “Compared to other machine-learning techniques, the generality of what it can accomplish continues to impress me,” says Chelsea Finn, who worked at the Berkeley lab and is now continuing that research at Stanford University in California.
This kind of learning gives an AI a much richer understanding of objects and the world in general, says Finn. If you had seen a water bottle or a sponge only in photographs, you might be able to recognize them in other images. But you would not really understand what they were or what they could be used for. “Your understanding of the world would be much shallower than if you could actually interact with them,” she says.
But this learning is a slow process. In a simulated environment, an AI can rattle through examples at lightning speed. In 2017, AlphaZero, the latest version of DeepMind’s self-taught game-playing software, was trained to become a superhuman player of Go, then chess and then shogi (a form of Japanese chess) in just over a day. In that time, it played more than 20 million training games of each event.
AI robots can’t learn this quickly. Almost all major results in deep learning have relied heavily on large amounts of data, says Jeff Mahler, co-founder of Ambidextrous, an AI and robotics company in Berkeley, California. “Collecting tens of millions of data points would cost years of continuous execution time on a single robot.” What’s more, the data might not be reliable, because the calibration of sensors can change over time and hardware can degrade.
Because of this, most robotics work that involves deep learning still uses simulated environments to speed up the training. “What you can learn depends on how good the simulators are,” says David Kent, a PhD student in robotics at the Georgia Institute of Technology in Atlanta. Simulators are improving all the time, and researchers are getting better at transferring lessons learnt in virtual worlds over to the real. Such simulations are still no match for real-world complexities, however.
Finn argues that learning using robots is ultimately easier to scale up than learning with artificial data. Her tool-using robot took a few days to learn a relatively simple task, but it did not require heavy monitoring. “You just run the robot and just kind of check in with it every once in a while,” she says. She imagines one day having lots of robots out in the world left to their own devices, learning around the clock. This should be possible — after all, this is how people gain an understanding of the world. “A baby doesn’t learn by downloading data from Facebook,” says Schmidhuber.
Learning from less data
A baby can also recognize new examples from just a few data points: even if they have never seen a giraffe before, they can still learn to spot one after seeing it once or twice. Part of the reason this works so quickly is because the baby has seen many other living things, if not giraffes, so is already familiar with their salient features.
A catch-all term for granting these kinds of abilities to AIs is transfer learning: the idea being to transfer the knowledge gained from previous rounds of training to another task. One way to do this is to reuse all or part of a pre-trained network as the starting point when training for a new task. For example, reusing parts of a DNN that has already been trained to identify one type of animal — such as those layers that recognize basic body shape — could give a new network the edge when learning to identify a giraffe.
An extreme form of transfer learning aims to train a new network by showing it just a handful of examples, and sometimes only one. Known as one-shot or few-shot learning, this relies heavily on pre-trained DNNs. Imagine you want to build a facial-recognition system that identifies people in a criminal database. A quick way is to use a DNN that has already seen millions of faces (not necessarily those in the database) so that it has a good idea of salient features, such as the shapes of noses and jaws. Now, when the network looks at just one instance of a new face, it can extract a useful feature set from that image. It can then compare how similar that feature set is to those of single images in the criminal database, and find the closest match.
Having a pre-trained memory of this kind can help AIs to recognize new examples without needing to see lots of patterns, which could speed up learning with robots. But such DNNs might still be at a loss when confronted with anything too far from their experience. It’s still not clear how much these networks can generalize.
Even the most successful AI systems such as DeepMind’s AlphaZero have an extremely narrow sphere of expertise. AlphaZero’s algorithm can be trained to play both Go and chess, but not both at once. Retraining a model’s connections and responses so that it can win at chess resets any previous experience it had of Go. “If you think about it from the perspective of a human, this is kind of ridiculous,” says Finn. People don’t forget what they’ve learnt so easily.
Learning how to learn
AlphaZero’s success at playing games wasn’t just down to effective reinforcement learning, but also to an algorithm that helped it (using a variant of a technique called Monte Carlo tree search) to narrow down its choices from the possible next steps10. In other words, the AI was guided in how best to learn from its environment. Chollet thinks that an important next step in AI will be to give DNNs the ability to write their own such algorithms, rather than using code provided by humans.
Supplementing basic pattern-matching with reasoning abilities would make AIs better at dealing with inputs beyond their comfort zone, he argues. Computer scientists have for years studied program synthesis, in which a computer generates code automatically. Combining that field with deep learning could lead to systems with DNNs that are much closer to the abstract mental models that humans use, Chollet thinks.
In robotics, for instance, computer scientist Kristen Grauman at Facebook AI Research in Menlo Park, California, and the University of Texas at Austin is teaching robots how best to explore new environments for themselves. This can involve picking in which directions to look when presented with new scenes, for instance, and which way to manipulate an object to best understand its shape or purpose. The idea is to get the AI to predict which new viewpoint or angle will give it the most useful new data to learn from.
Researchers in the field say they are making progress in fixing deep learning’s flaws, but acknowledge that they’re still groping for new techniques to make the process less brittle. There is not much theory behind deep learning, says Song. “If something doesn’t work, it’s difficult to figure out why,” she says. “The whole field is still very empirical. You just have to try things.”
For the moment, although scientists recognize the brittleness of DNNs and their reliance on large amounts of data, most say that the technique is here to stay. The realization this decade that neural networks — allied with enormous computing resources — can be trained to recognize patterns so well remains a revelation. “No one really has any idea how to better it,” says Clune.
Nature 574, 163-166 (2019)
doi: 10.1038/d41586-019-03013-5
References
1.
Eykholt, K. et al. IEEE/CVF Conf. Comp. Vision Pattern Recog. 2018, 1625–1634 (2018).
ArticleGoogle Scholar
2.
Finlayson, S. G. et al. Science 363, 1287–1289 (2019).
PubMedArticleGoogle Scholar
3.
Elsayed, G. F., Goodfellow, I. & Sohl-Dickstein, J. Preprint at https://arxiv.org/abs/1806.11146 (2018).
4.
Szegedy, C. et al. Preprint at https://arxiv.org/abs/1312.6199v1 (2013).
5.
Nguyen, A., Yosinski, J. & Clune, J. IEEE Conf. Comp. Vision Pattern Recog. 2015, 427–436 (2015).
ArticleGoogle Scholar
6.
Alcorn, M. A. et al. IEEE Conf. Comp. Vision Pattern Recog. 2019, 4845–4854 (2019).
Google Scholar
7.
Hendrycks, D., Zhao, K., Basart, S., Steinhardt, J. & Song, D. Preprint at https://arxiv.org/abs/1907.07174 (2019).
8.
Huang, S., Papernot, N., Goodfellow, I., Duan, Y. & Abbeel, P. Preprint at https://arxiv.org/abs/1702.02284 (2017).
9.
Gleave, A. et al. Preprint at https://arxiv.org/abs/1905.10615 (2019).
10.
Silver, D. et al. Science 362, 1140–1144 (2018).
PubMedArticleGoogle Scholar
Download references
show more
Latest on:
Computer science
Information technology
Quantum computing takes flight
Quantum computing takes flight
NEWS & VIEWS 23 OCT 19
Hello quantum world! Google publishes landmark quantum supremacy claim
Hello quantum world! Google publishes landmark quantum supremacy claim
NEWS 23 OCT 19
The social-media war, reclaiming classics from the alt-right, and a fusion of physics and dance: New in paperback
The social-media war, reclaiming classics from the alt-right, and a fusion of physics and dance: New in paperback
BOOKS & ARTS 15 OCT 19
https://www.nature.com/articles/d41586-019-03013-5
投稿コメント全ログ コメント即時配信 スレ建て依頼 削除コメント確認方法

すべてのページの引用、転載、リンクを許可します。確認メールは不要です。引用元リンクを表示してください。