http://www.asyura2.com/16/health18/msg/704.html
Tweet |
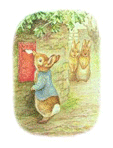
9時5時勤務は夜型に不利、脳の働きに影響も=英研究
2019/02/18
BBC News
早起きして活動する「朝型」の人と夜に活動的になる「夜型」の人では、学校や職場の稼働時間の脳の働きが異なることが、イギリスの大学の研究で明らかになった。
学術誌「Sleep」に掲載されたこの研究では、午前2時半に寝て午前10時15分に起きる夜型の人と、午後11時前に寝て午前6時半に起きる朝型の人の脳をスキャンして働きを比べた。
その結果、午前8時から午後8時までの時間帯では、夜型の人の脳内では集中力を司る領域の活動が少ないことが分かった。また注力が散漫になる、反応が遅くなる、眠気といった傾向がみられた。
研究チームは、夜型の人は典型的な勤務時間の「制約」で不利な状態にあると指摘した。
学校や職場の稼働時間は、夜型の人にとって体の自然なサイクルではない。研究チームは、こうした状況が健康に与える影響をもっと調査する必要があると呼びかけた。
「体内リズムと戦っている」
研究ではまず、朝型と夜型、計38人の休息時の脳の働きを核磁気共鳴画像法(MRI)で測定した。
その後、午前8時から午後8時にさまざまなテストをこなしてもらい、眠気の度合いを報告してもらった。
朝型の人たちは朝早い時間のテストで最も眠気が少なく、反応も速かった。またこの時間帯、夜型の人よりもテストの成績が格段に上だった。
一方、夜型の人たちが最も眠気が少なく、反応が早かったのは午後8時台だった。しかしテストの成績は、この時間帯でも朝型の人と比べて非常に良いということではなかった。
朝型の人たちは、良い成績と眠気の少なさを示す脳の領域が、常に極めて活発だった。このことから研究チームは、夜型の人の場合は、典型的な日中の勤務時間中には同じ脳の領域の神経結合が阻害されている可能性があると指摘した。
研究を主導した英バーミンガム大学人間脳健康センターのエリース・フェイサー=チャイルズ博士は、こうした結果が出たのは「夜型の人はずっと、自分本来の生活リズムを曲げて生きているからかもしれない」と話した。
「夜型の人は学校に行くために早起きし、その後も仕事のために早起きする。日常的に個人的な好みや体内リズムと戦わなければならない」
その上で、学校や職場の稼働時間に合わせることが夜型の人たちの健康や生産性にどう影響しているかを理解するのは、「きわめて重要」だと述べた。
研究者によると、人類の40〜50%は遅い時間に寝て午前8時20分以降に起きるのを好んでいるという。
「午前9時から午後5時という典型的な平日の稼働時間だと、夜型の人の午前中の生産性は落ち、脳の注意力を司る領域の神経結合が減り、日中の眠気を引き起こす可能性がある」と、フェイサー=チャイルズ博士は指摘する。
「時間をもっと柔軟に管理できる社会であれば、生産性を高めると同時に健康リスクを下げるという長い道のりを歩むことができるかもしれない」
フェイサー=チャイルズ博士によると、この研究で明らかになった脳の神経結合の違いは損傷ではなく、回復可能なものだという。
一方で、この研究の限界も指摘されている。
この調査では午後の脳の働きを測定していない。またライフスタイルの違いなど、研究では取り上げられていない要因が結果を左右している可能性もある。
キングス・コレッジ・ロンドンの脳神経学者アレックス・ネズビット博士は、この研究によって人の脳の働きは時間帯だけでなく体内時計にも左右されるという証拠が新たに増えたと話す。
「多くの人が『9時5時』のルーティンを課せられている中、こうした要因が重要だということがどんどん明らかになっている」
研究チームは、朝型と夜型の違いが脳のほかの領域に与える影響についてもさらなる研究が必要だと述べている。
(英語記事 'Owls' and 'larks' brains differ - study )
提供元:https://www.bbc.com/japanese/features-and-analysis-47274812
9時5時勤務は夜型に不利、脳の働きに影響も=英研究
2019/02/18
BBC News
早起きして活動する「朝型」の人と夜に活動的になる「夜型」の人では、学校や職場の稼働時間の脳の働きが異なることが、イギリスの大学の研究で明らかになった。
学術誌「Sleep」に掲載されたこの研究では、午前2時半に寝て午前10時15分に起きる夜型の人と、午後11時前に寝て午前6時半に起きる朝型の人の脳をスキャンして働きを比べた。
その結果、午前8時から午後8時までの時間帯では、夜型の人の脳内では集中力を司る領域の活動が少ないことが分かった。また注力が散漫になる、反応が遅くなる、眠気といった傾向がみられた。
研究チームは、夜型の人は典型的な勤務時間の「制約」で不利な状態にあると指摘した。
学校や職場の稼働時間は、夜型の人にとって体の自然なサイクルではない。研究チームは、こうした状況が健康に与える影響をもっと調査する必要があると呼びかけた。
「体内リズムと戦っている」
研究ではまず、朝型と夜型、計38人の休息時の脳の働きを核磁気共鳴画像法(MRI)で測定した。
その後、午前8時から午後8時にさまざまなテストをこなしてもらい、眠気の度合いを報告してもらった。
朝型の人たちは朝早い時間のテストで最も眠気が少なく、反応も速かった。またこの時間帯、夜型の人よりもテストの成績が格段に上だった。
一方、夜型の人たちが最も眠気が少なく、反応が早かったのは午後8時台だった。しかしテストの成績は、この時間帯でも朝型の人と比べて非常に良いということではなかった。
朝型の人たちは、良い成績と眠気の少なさを示す脳の領域が、常に極めて活発だった。このことから研究チームは、夜型の人の場合は、典型的な日中の勤務時間中には同じ脳の領域の神経結合が阻害されている可能性があると指摘した。
研究を主導した英バーミンガム大学人間脳健康センターのエリース・フェイサー=チャイルズ博士は、こうした結果が出たのは「夜型の人はずっと、自分本来の生活リズムを曲げて生きているからかもしれない」と話した。
https://academic.oup.com/sleep/advance-article/doi/10.1093/sleep/zsz033/5316210
「夜型の人は学校に行くために早起きし、その後も仕事のために早起きする。日常的に個人的な好みや体内リズムと戦わなければならない」
その上で、学校や職場の稼働時間に合わせることが夜型の人たちの健康や生産性にどう影響しているかを理解するのは、「きわめて重要」だと述べた。
研究者によると、人類の40〜50%は遅い時間に寝て午前8時20分以降に起きるのを好んでいるという。
「午前9時から午後5時という典型的な平日の稼働時間だと、夜型の人の午前中の生産性は落ち、脳の注意力を司る領域の神経結合が減り、日中の眠気を引き起こす可能性がある」と、フェイサー=チャイルズ博士は指摘する。
「時間をもっと柔軟に管理できる社会であれば、生産性を高めると同時に健康リスクを下げるという長い道のりを歩むことができるかもしれない」
フェイサー=チャイルズ博士によると、この研究で明らかになった脳の神経結合の違いは損傷ではなく、回復可能なものだという。
一方で、この研究の限界も指摘されている。
この調査では午後の脳の働きを測定していない。またライフスタイルの違いなど、研究では取り上げられていない要因が結果を左右している可能性もある。
キングス・コレッジ・ロンドンの脳神経学者アレックス・ネズビット博士は、この研究によって人の脳の働きは時間帯だけでなく体内時計にも左右されるという証拠が新たに増えたと話す。
「多くの人が『9時5時』のルーティンを課せられている中、こうした要因が重要だということがどんどん明らかになっている」
研究チームは、朝型と夜型の違いが脳のほかの領域に与える影響についてもさらなる研究が必要だと述べている。
(英語記事 'Owls' and 'larks' brains differ - study )
提供元:https://www.bbc.com/japanese/features-and-analysis-47274812
https://academic.oup.com/sleep
Circadian phenotype impacts the brain’s resting state functional connectivity, attentional performance and sleepiness
Elise R Facer-Childs Brunno M Campos Benita Middleton Debra J Skene Andrew P Bagshaw
Sleep, zsz033, https://doi.org/10.1093/sleep/zsz033
Published: 15 February 2019 Article history
Split View PDF Cite
Permissions
Share
Abstract
INTRODUCTION
Functional connectivity (FC) of the human brain’s intrinsically connected networks underpins cognitive functioning and disruptions of FC are associated with sleep and neurological disorders. However, there is limited research on the impact of circadian phenotype and time of day on FC.
STUDY OBJECTIVES
The aim of this study was to investigate resting state FC of the default mode network (DMN) in Early and Late circadian phenotypes over a socially constrained day.
METHODS
38 healthy individuals (14 male, 22.7 ± 4.2 years) categorised as Early (n =16) or Late (n = 22) using the Munich ChronoType Questionnaire took part. Following a two week baseline of actigraphy coupled with saliva samples for melatonin and cortisol rhythms, participants underwent testing at 14.00 h, 20.00 h and 08.00 h the following morning. Testing consisted of resting state functional MRI, a structural T1 scan, attentional cognitive performance tasks and self-reported daytime sleepiness. Seed based FC analysis from the medial prefrontal and posterior cingulate cortices of the DMN was performed, compared between groups and linked with behavioural data.
RESULTS
Fundamental differences in the DMN were observed between Early and Late circadian phenotypes. Resting state FC of the DMN predicted individual differences in attention and subjective ratings of sleepiness.
CONCLUSION
Differences in FC of the DMN may underlie the compromised attentional performance and increased sleepiness commonly associated with Late types when they conform to a societally constrained day that does not match their intrinsic circadian phenotype.
Resting-state functional magnetic resonance imaging (fMRI), circadian phenotype, sleep, default mode network, attentional performance, sleepiness, circadian rhythms
Topic: phenotype sleep drowsiness functional magnetic resonance imaging cognitive ability
Issue Section: Original Article
PDF
This content is only available as a PDF.
c Sleep Research Society 2019. Published by Oxford University Press [on behalf of the Sleep Research Society].
This is an Open Access article distributed under the terms of the Creative Commons Attribution License (http://creativecommons.org/licenses/by/4.0/), which permits unrestricted reuse, distribution, and reproduction in any medium, provided the original work is properly cited.
Article has an altmetric score of 103
View Metrics
Email alerts
New issue alert
Advance article alerts
Article activity alert
Subject alert
Receive exclusive offers and updates from Oxford Academic
Related articles in
Google Scholar
Related articles in PubMed
Congenital Hypopituitarism: Various Genes, Various Phenotypes.
Diffuse optical tomography for the detection of perinatal stroke at the cot side: a pilot study.
The Effects of Probiotics on Symptoms of Depression: Protocol for a Double-Blind Randomized Placebo-Controlled Trial.
Corrigendum: Predicting spirometry readings using cough sound features and regression (2018 Physiol. Meas. 39 095001).
Citing articles via
Google Scholar
CrossRef
Latest
Most Read Most Cited
Factors involved in sleep efficiency: a population-based study of community-dwelling elderly persons
Circadian phenotype impacts the brain’s resting state functional connectivity, attentional performance and sleepiness
Differential Effects of Split and Continuous Sleep on Neurobehavioral Function and Glucose Tolerance in Sleep-Restricted Adolescents
Categorization deficit of facially expressed anger in insomnia (Commentary on Zhang et al. Individuals with insomnia misrecognize angry faces as fearful faces while missing the eyes: an eye-tracking study)
Objective data contribute to the school start time debate
Accepted Manuscript
c Sleep Research Society 2019. Published by Oxford University Press [on behalf of the Sleep
Research Society].
This is an Open Access article distributed under the terms of the Creative Commons Attribution
License (http://creativecommons.org/licenses/by/4.0/), which permits unrestricted reuse,
distribution, and reproduction in any medium, provided the original work is properly cited.
Title: Circadian phenotype impacts the brain’s resting state functional connectivity,
attentional performance and sleepiness
Authors: Elise R. Facer-Childs1,2,5*, Brunno M. Campos3
, Benita Middleton4
, Debra J.
Skene4
, Andrew P. Bagshaw2,5
Affiliations:
1
School of Biosciences, University of Birmingham, Birmingham, B15 2TT, UK
2Centre for Human Brain Health, University of Birmingham, Birmingham, B15 2TT, UK
3
School of Medical Sciences, University of Campinas, Campinas - SP, 13083-970, Brazil
4
Faculty of Health & Medical Sciences, University of Surrey, Guildford, GU2 7XH, UK
5
School of Psychology, University of Birmingham, Birmingham, B15 2TT, UK
*Correspondence:
Dr Elise R. Facer-Childs
E.R.Facer-Childs@bham.ac.uk
Downloaded from https://academic.oup.com/sleep/advance-article-abstract/doi/10.1093/sleep/zsz033/5316210 by Ritsumeikan University user on 18 February 2019
Accepted Manuscript
2
Abstract
INTRODUCTION: Functional connectivity (FC) of the human brain’s intrinsically connected
networks underpins cognitive functioning and disruptions of FC are associated with sleep and
neurological disorders. However, there is limited research on the impact of circadian phenotype and
time of day on FC.
STUDY OBJECTIVES: The aim of this study was to investigate resting state FC of the default mode
network (DMN) in Early and Late circadian phenotypes over a socially constrained day.
METHODS: 38 healthy individuals (14 male, 22.7 ± 4.2 years) categorised as Early (n =16) or Late (n
= 22) using the Munich ChronoType Questionnaire took part. Following a two week baseline of
actigraphy coupled with saliva samples for melatonin and cortisol rhythms, participants underwent
testing at 14.00 h, 20.00 h and 08.00 h the following morning. Testing consisted of resting state
functional MRI, a structural T1 scan, attentional cognitive performance tasks and self-reported
daytime sleepiness. Seed based FC analysis from the medial prefrontal and posterior cingulate
cortices of the DMN was performed, compared between groups and linked with behavioural data.
RESULTS: Fundamental differences in the DMN were observed between Early and Late circadian
phenotypes. Resting state FC of the DMN predicted individual differences in attention and subjective
ratings of sleepiness.
CONCLUSION: Differences in FC of the DMN may underlie the compromised attentional
performance and increased sleepiness commonly associated with Late types when they conform to a
societally constrained day that does not match their intrinsic circadian phenotype.
Key words: Resting-state functional magnetic resonance imaging (fMRI), circadian phenotype, sleep,
default mode network, attentional performance, sleepiness, circadian rhythms
Statement of significance: Misalignment between an individual’s biological timing and behaviour
(e.g. as a result of shift-work or jet lag) has adverse impacts on brain function, performance and
health. We found that people with a late sleep-wake preference, often called ‘night owls’, have
significantly lower functional connectivity in the brain’s ‘default mode network’, which is involved in
maintenance of consciousness and a range of cognitive functions. Importantly, these differences at
rest were predictive of poorer attentional performance (slower reaction time), and increased subjective
sleepiness. This may represent an intrinsic neuronal mechanism, which leads to ‘night owls’ being
comprised during a normal working day. Future work needs to account for these differences, while
targeting sleep/circadian biology could aid in improving health and performance.
Downloaded from https://academic.oup.com/sleep/advance-article-abstract/doi/10.1093/sleep/zsz033/5316210 by Ritsumeikan University user on 18 February 2019
Accepted Manuscript
3
Introduction
It is estimated that nearly 70 million individuals in the US alone suffer from some sort of disturbance
to the sleep/wake axis which impedes normal functioning and has potentially damaging effects on
health and well-being.
1
Societal demands are often in conflict with an individual’s endogenous
biological rhythms, leading to adverse impacts on mental and physical health as well as performance.
An extreme example of this is shift work, whereby misalignment between an externally imposed
work/rest schedule and internal circadian timing can lead to cognitive deficits,
2
poorer mental health,
3
increased health risks including cancer4
and a compromised immune system.
5
However, misalignment does not have to be driven by unusual work schedules. By definition, the
important issue is that one’s internal temporal organisation (i.e. circadian phenotype) and external
schedule are in conflict. In particular, a standard working day of 09:00 - 17:00 h may be detrimental
for an individual whose biological preference is for a late sleep-wake cycle. Compounding the
problem, misalignment can also be associated with a cumulative sleep debt, as sleep is curtailed
because of late sleep onset, with a similar type and range of adverse outcomes.
6 This aspect of
misalignment is much less understood than night shift work, but potentially of greater importance
given that, according to estimates from the Office of National Statistics, 12 % of the population work
night shifts, whereas around 50 % have a late preference favouring a wake up time later than 08:18
am.
7 Therefore, there is a critical need to increase our understanding of these issues in order to
minimise health risks in society and maximise productivity.
It is well established that there are individual differences in circadian timing, i.e. diurnal preference8
and chronotype.
7 At the extreme end of the continuum, these different groups of individuals can be
identified as ‘larks’ or ‘owls’ (referred to here as Early (ECP) and Late (LCP) circadian phenotypes
based on objective actigraphy and circadian phase markers). Compared to LCPs, ECPs have less
disrupted sleep,
9 make healthier food choices,
10 thereby minimising risks of obesity and diabetes,
11
and reach higher standards in the sports world.
12 Conversely, LCPs have been linked to greater
daytime sleepiness,
13 increased alcohol consumption and substance abuse,
14 decreased psychological
well-being through higher rates of depression,
15 sleep disorders,
16 negative health outcomes,
17 and
have even been linked to higher mortality rates.
18 Constant desynchronisation of their internal
circadian rhythms through trying to ‘fit in’ to external societal time e.g. work/school schedules has
been suggested as the root cause of these adverse impact on LCPs. This mismatch of biological and
social time has been called ‘social jetlag’.
19
The consequences of sleep and circadian disruption on health and cognitive performance are well
established. The application of fMRI in this area is still relatively sparse and much of the literature
surrounding the relationship between brain function and attention has been focused on task-based
fMRI. However, optimal cognitive performance and good mental health rely upon the appropriate
coordination of activity between distributed intrinsic functional neuronal networks (often referred to
as intrinsically connected networks, ICNs). One ICN, the default mode network (DMN), is
particularly affected by sleep onset,
20 sleep deprivation,
21 variations in habitual sleep patterns across
individuals,
22 and exhibits diurnal variation in its functional connectivity (FC).
23 The DMN is most
active in the absence of external cognitive demand,
24 and has been associated with functions as
diverse as self-referential processing25 maintaining consciousness,
26 regulating cognition,
27 attention,
28
and working memory.
29 It is also modified in a range of psychiatric and neurological disorders,
30
including Alzheimer’s disease31 and depression.
32
Downloaded from https://academic.oup.com/sleep/advance-article-abstract/doi/10.1093/sleep/zsz033/5316210 by Ritsumeikan University user on 18 February 2019
Accepted Manuscript
4
Resting state fMRI provides a complementary approach to task-based fMRI, with the efficiency and
integrity of ICNs been linked to intellectual performance33 and greater intelligence,34 marking the
importance that testing resting state FC (rs-FC) could play in predicting measures of cognitive
function. Only a handful of studies have explored the link between FC, sleep, circadian phenotype and
cognitive performance.35, 36 However, these investigations used task-based fMRI and controlled for
the effect of circadian phenotype by scheduling testing based on internal biological time e.g. every 4 h
starting 1.5 h after waking, preventing the exploration of the effect of circadian phenotype in real-life
throughout a typical societally constrained day.
In summary, neuroimaging is increasingly used as a technique in sleep research, but inter-subject
variability e.g. circadian phenotype brings another level of complexity that is rarely accounted for,
despite emerging research showing diurnal variation in brain function.
23, 37 Given that the DMN is
evidently vital to basic maintenance of consciousness, affected by sleep alterations, and plays a role in
cognitive functioning, it was used as the network of interest in the present study to examine the
impact of circadian phenotype on resting state brain function during the course of a typical societally
constrained day (08:00 h to 20:00 h). Both anterior (medial prefrontal cortex, mPFC) and posterior
(posterior cingulate cortex, PCC) regions of the DMN were used as seed regions to gather information
about the functional integrity of the DMN at rest, and these data linked to attentional performance and
sleepiness outside of the MRI scanner. We hypothesised that LCPs would show disrupted FC
compared to LCPs, and that FC differences would be correlated with behaviour.
Methods
Participants
The study was approved by the University of Birmingham Research Ethics Committee. Individuals (n
= 204) from the University of Birmingham and surrounding community completed the Munich
Chronotype Questionnaire (MCTQ38) and were screened for any contraindications to inclusion in the
study based on medical history and magnetic resonance safety. Exclusion criteria were; 1) no prior or
current diagnoses of sleep, neurological or psychiatric disorders; 2) taking medications that affect
sleep or melatonin/cortisol rhythms and; 3) intermediate chronotype indicated by corrected mid-sleep
times on free days (MSFsc) from the MCTQ.
A total of 38 healthy individuals (14 male, 22.7 ± 4.2 years) who were categorised as ‘Early’
(n = 16, age 24.7 ± 4.6 years, nine female, MSFsc 02:24 ± 00:10 h) or ‘Late’ (n = 22, age 21.3
± 3.3 years, 15 female, MSFsc 06:52 ± 00:17 h) chronotypes and who also passed all
inclusion criteria were invited to take part in the main study. Participants gave written
informed consent before involvement and all details provided were given on a voluntary
basis. After completing questionnaires, physiological sampling and between 13-16 days of
actigraphy in their home environment (details below), participants attended the Birmingham
University Imaging Centre for testing sessions at 14:00 h, 20:00 h and 08:00 h (GMT) the
Downloaded from https://academic.oup.com/sleep/advance-article-abstract/doi/10.1093/sleep/zsz033/5316210 by Ritsumeikan University user on 18 February 2019
Accepted Manuscript
5
following morning. Individuals went home in between testing sessions. Testing sessions were
conducted in a specific order (14:00 h, 20:00 h and 08:00 h) to prevent the 14:00 h and 20:00
h sessions being affected by sleep deprivation. This design allowed all individuals to wake up
naturally for the 14:00 h and 20:00 h. Summary details of participants’ data can be found in
Table 1. At each testing session participants underwent a resting state fMRI and T1 scan
followed by cognitive testing (psychomotor vigilance and Stroop tasks) and subjective
sleepiness ratings (details below). As part of the cognitive testing that was completed at each
session, a questionnaire was developed and administered to gather details about what was
occurring between sessions when participants left the laboratory. In an attempt to partially
control for external variables and confirm no differences between the groups, information
gathered included hours since; 1) food intake; 2) caffeine consumption; 3) exercise; 4)
exposure to natural light and 5) exposure to indoor light (Table 1).
Sleep Analysis
Actigraphs (ActiwatchR Light, AWLs, 2006, Cambridge Neurotechnology Ltd) were worn on
participants’ non-dominant wrist to gather activity and light exposure data (1-32,000 lux) for 13-16
days prior to testing sessions. This allowed sleep and activity patterns to be monitored continuously in
the home environment. Data were acquired in 1-minute epochs (medium sensitivity setting),
confirmed with daily sleep diaries, and analysed using Sleep Analysis 7 Software (version 7.23,
Cambridge Neurotechnology Ltd). Throughout this period participants were following preferred
routines and were not confined to particular schedules.
Physiological Data
Saliva samples were provided during one morning and one evening the week of testing by spitting
into pre-labelled polypropylene collection tubes (7ml plastic bijou) following strict standardised
protocols. Participants were trained in how to take the saliva samples in their home environment
during their initial set up visit and the protocol instructions were discussed to ensure participants
understood what was required. In addition, a sample collection record sheet was attached to both
morning and evening protocols to ensure that the exact times samples were taken could be recorded.
During the sampling periods, participants were asked to abstain from caffeinated drinks, alcoholic
drinks or any drinks containing artificial colouring. They were also asked to refrain from cleaning
their teeth, chewing gum or going to the bathroom at least 15 minutes before each sample. Evening
samples were collected from a seated position whilst in dim lighting conditions (no overhead lights,
no electronic screens and curtains closed) every 30 minutes from three hours prior to individual
Downloaded from https://academic.oup.com/sleep/advance-article-abstract/doi/10.1093/sleep/zsz033/5316210 by Ritsumeikan University user on 18 February 2019
Accepted Manuscript
6
habitual bedtime until one hour after. Morning samples were collected on awakening, every 15
minutes for the first hour and every 30 minutes for the following two hours. All samples were
anonymised. Radioimmunoassays (RIA) of melatonin and cortisol were performed (Stockgrand Ltd,
University of Surrey) using an Iodine-125 radioactive labelled tracer and solid phase separation.
39
Assays were run with quality controls (QCs) before and after samples. These QC values were then
averaged to give one value per assay to calculate inter-assay coefficients of variation (CV %). The
limit of detection for the melatonin assay was 0.72 ± 0.08 pg/ml and CVs were 9.4 % at 44.4 pg/ml,
9.9 % at 20.1 pg/ml and 12.2 % at 9.0 pg/ml (n = 13 at each concentration). The limit of detection for
the cortisol assay was 0.45 ± 0.06 nmol/L and inter-assay CVs were 8.3 % at 48.0 nmol/l, 6.1 % at
15.9 nmol/l and 9.8 % at 3.0 nmol/l (n = 15 at each concentration).
Individual dim light melatonin onset (DLMO) values were calculated using the mean of the individual
baseline concentration values plus two standard deviations of the mean. Due to intra-subject
variability in melatonin concentrations these calculations were performed relative to each individual.
This concentration was used to calculate the timing of melatonin onset through a linear response
function. The peak time of the cortisol awakening response was calculated as the time of highest
cortisol concentration recorded. All results were calculated based on individual sample timings taken
from sample collection record sheets. Due to insufficient or contaminated samples, DLMO values
were unable to be calculated for two ECPs and four LCPs.
Neuroimaging Acquisition
Imaging data were acquired using a Philips Achieva 3T MRI scanner with a 32-channel head coil.
Whole brain coverage gradient echo-planar imaging data were acquired parallel to the AC-PC line
with the following parameters: 15 minutes, 450 volumes, TR = 2000 ms, TE = 35 ms, flip angle = 80?,
3 x 3 x 4 mm voxels, 32 slices, no gap, matrix = 80 x 80 x 32. Standard high resolution 3D anatomical
T1-weighted scans (sagittal acquisition, TR = 8.4 ms, TE = 3.8 ms, flip angle = 8?, 1 mm isotropic
voxel, matrix = 288 x 288 x 175) were also collected to facilitate co-registration. Respiratory and
cardiac fluctuations were recorded with the pulse oximeter and pneumatic belt provided by the
scanner manufacturer. A camera was placed in the scanner during each session to monitor
participants’ eyes, confirm they remained open and that sleep had not been initiated. If eye closure
exceeded 15 s, which is half a 30 s epoch according to the standard sleep staging approach,
40 the scan
was re-started. This occurred in one scan for one participant. Standard Birmingham University
Imaging Centre operating procedures were followed for the MRI safety screening and during the
scanning sessions, and participants were not asked to perform any task.
Neuroimaging Pre-processing
FMRI preprocessing and analysis was performed using UF2C,
41 PhysIO,
42 and SPM1243 toolboxes
implemented in MATLAB (MathWorks, USA). Preprocessing was carried out in UF2C using
standardised methodologies implemented in SPM12. Data were re-orientated to the anterior
commissure as origin, motion corrected using rigid body transformations (three translational and three
rotational planes), spatially normalised (MNI-152 template space), spatially smoothed with a 6 mm
Gaussian kernel and detrended (temporal linear trends removal). Physiological noise corrections
(RETROICOR for a 3rd order cardiac, 4th order respiratory, and 1st order interaction Fourier expansion
of cardiac and respiratory phase, heart rate variability and respiratory volume per time) were modelled
using the PhysIO toolbox. This resulted in 18 nuisance regressors which were added to preprocessing
Downloaded from https://academic.oup.com/sleep/advance-article-abstract/doi/10.1093/sleep/zsz033/5316210 by Ritsumeikan University user on 18 February 2019
Accepted Manuscript
7
routines in UF2C, along with average signals for white matter (WM) and cerebro-spinal fluid (CSF)
and six movement (three translational and three rotational) regressors. High-pass (> 0.008 Hz) and
low-pass (< 0.1 Hz) temporal filtering was applied to remove confounding physiological frequencies.
Framewise displacement (FD) and derivative variance (DVARs) were calculated,
44, 45 and any scan
with an average FD value above 0.5 mm was excluded. This resulted in one scan (ECP, 14:00 h)
being removed from further analysis. Head movement (translational, rotational, FD and DVARS) did
not differ significantly between the groups or between times of day.
Neuroimaging Analysis
A seed-based FC approach was used to analyse the data using predefined seeds for the frontal (mPFC)
and posterior (PCC) regions of the DMN.
46 Pearson correlation maps were then converted to z-score
maps using Fisher’s Transformation. Using the general linear model (GLM) implemented in SPM12,
second level group analyses were performed using a flexible factorial design. The second level
analyses were performed using a voxel-level threshold FWE corrected at p < 0.05. A subsequent
extent threshold (FWE corrected at p < 0.05) was used to concentrate on the significant results at the
cluster-level. Subject, group and time of day were added as factors and the model was set up for the
main effect of group (ECPs and LCPs), the main effect of time of day (morning; 08:00 h, afternoon;
14:00 h and evening: 20:00 h) as well as the interaction of group and time of day. All subject
variability including age and gender were accounted for as covariates by adding subject as a factor.
Descriptions of significant findings from the mPFC seed (voxel-level threshold FWE corrected at p <
0.05, with a subsequent extent threshold of 100 voxels) and PCC seed (voxel-level threshold FWE
corrected at p < 0.05, with a subsequent extent threshold of 150 voxels), are presented as total voxels,
peak t score and peak MNI centroid cluster coordinates [x y z]. Extent thresholds were selected as a
fifth of the biggest cluster. All significant areas were transformed in a binary mask and the z-scored
values from the correlation map within this mask were averaged generating a single value
representing average rs-FC across all significant clusters per participant for each scan. These values
were used to explore the predictive effects of rs-FC on attention and daytime sleepiness using
generalised estimating equations (details given in Statistical Analysis section).
Attentional Performance & Sleepiness
Following the scan, participants were immediately taken to a testing room where a two-minute
psychomotor vigilance task (PVT)
47 and a Stroop Colour-Word Task48 were completed. A visual
version of the Stroop test was used which consisted of 60 trials with equal proportion of congruent
and incongruent stimuli (30 of each). Presentation time was not fixed i.e. stimuli were visible until
response. Reaction time values were used from the PVT and the Stroop task (averaged correct
congruent and incongruent trials) as indices of attentional performance. Incompletion of the Stroop
test resulted in one participant’s results being excluded for further analysis. Daytime sleepiness,
measured using the Karolinska Sleepiness Scale (KSS),
49 was completed before the cognitive tests
were performed.
Statistical Analysis
Downloaded from https://academic.oup.com/sleep/advance-article-abstract/doi/10.1093/sleep/zsz033/5316210 by Ritsumeikan University user on 18 February 2019
Accepted Manuscript
8
Statistical comparisons of behavioural data were performed in GraphPad Prism (version 7, La Jolla,
USA) and SPSS (IBM SPSS Statistics, version 24, Chicago) using two sided unpaired t-tests, MannWhitney U tests, Fisher’s exact test and linear regression after testing for equality of means with
Levene’s test. All p-values were FDR corrected to control for multiple comparisons.50 Diurnal
variations in performance and sleepiness variables were plotted using second order regression curves
and analysed using two-way analysis of variance (ANOVA) for repeated measures with post hoc
multiple comparison tests. Non-parametric tests were implemented where data did not follow a
normal distribution.
To explore the predictive effects of rs-FC on performance variables and daytime sleepiness an
extension of the generalised linear model (generalised estimating equations, GEEs) were used in
SPSS. GEEs account for repeated measures and within subject variability and do not assume normal
distributions or linear relationships. GEEs are often used in studies with time of day data to model the
average effect, and have been used in sleep and circadian research to model the relationship between
insomnia, depression and chronotype16 as well as in studies on sleep durations51, 52 and circadian
patterns in epilepsy.
53 Data used in GEE analyses were z-scored average rs-FC values across all
clusters for each participant, individual reaction times (PVT and Stroop) and KSS score. A scale
linear response GEE with identity link function for scale data was used to model the independent
effects of rs-FC on attentional performance. A negative binomial GEE with log link function for count
data was used to model the effects of rs-FC on sleepiness. Both models were designed adding Subject
ID as a subject variable, and circadian phenotype (ECP/LCP) and time of day (08:00 h, 14:00 h and
20:00 h) as within-subject variables. Time of day was also added as a fixed factor. When interaction
terms were not significant they were removed from the model and the analysis re-run. Corrected quasi
likelihood under independence model criterion (QICC) values were used to choose the best fit for
models.
Significance levels are displayed as not significant (ns), p < 0.05 (*), p < 0.01 (**), p < 0.001 (***)
and p < 0.0001 (****). Exact p values are given apart from when significance is identified as less than
0.0001, in which case p < 0.0001 is reported. Results are shown using the mean ± standard error of the
mean (SEM) unless specified otherwise.
Results
Circadian Phenotyping
Individuals were initially categorised into Early (n = 16) and Late (n = 22) chronotypes using MSFsc,
calculated using the MCTQ.
38 These groups were confirmed as ECPs and LCPs by analysis of
biological circadian phase markers, namely DLMO and time of peak morning concentration of the
cortisol awakening response, in addition to sleep start and wake up times calculated from actigraphy
analysis. All parameters were significantly different between the groups, occurring approximately 3.5
h ? 4.5 h earlier in ECPs than LCPs (Table 1). MSFsc was ~4 h earlier in ECPs compared to LCPs
(t(36) = 12.2, p < 0.0001). DLMO and peak time of morning cortisol also differed significantly by
~3.5 h and ~4 h respectively (t(30) = 6.8, p < 0.0001 and t(36) = 8.0, p < 0.0001). These results were
consistent with sleep onset and wake up times calculated from actigraphy data, with a difference
between the groups of ~3.5 h (t(34) = 8.9, p < 0.0001 and t(34) = 9.9, p < 0.0001).
Downloaded from https://academic.oup.com/sleep/advance-article-abstract/doi/10.1093/sleep/zsz033/5316210 by Ritsumeikan University user on 18 February 2019
Accepted Manuscript
9
Each of these parameters was significantly correlated with MSFsc (Figure 1). Significant linear
regressions were found between MSFsc and DLMO (R2
= 0.65, p < 0.0001), peak time of cortisol
awakening response (R2
= 0.75, p < 0.0001), sleep onset (R2
= 0.80, p < 0.0001) and wake up time (R2
= 0.86, p < 0.0001). All other actigraphic parameters were not significantly different between ECPs
and LCPs (Table 1). As all participants in this study were following their preferred schedules for the
duration of the experiment, these findings confirmed that neither group were acutely sleep deprived
during the baseline period. However, in order to rule out a baseline sleep debt effect, additional
analyses were run to examine the relationships between sleep efficiency and rs-FC. No significant
correlations were found. These results support the classification into circadian phenotypes and
demonstrate that these two groups are behaviourally and physiologically different in sleep timings and
circadian phase but not in other sleep parameters.
***INSERT TABLE 1***
***INSERT FIGURE 1***
Resting state functional connectivity in circadian phenotypes
Whole group analyses showed a clear DMN from both seeds, with significant FC (FWE corrected p <
0.05) observed between all major components of the DMN including the PCC/precuneus, mPFC,
bilateral angular and temporal gyri, and cerebellum (Figure 2, grayscale underlay).
The flexible factorial model showed clear significant differences between circadian phenotype groups
but no significant main effect of time of day (Figure 2). ECPs had significantly increased FC
compared to LCPs at all times of day in 15 of the total 18 supra-threshold clusters identified from
both seeds (FWE corrected at p < 0.05). When seeding in the PCC, there was significantly higher FC
for ECPs from PCC to the precuneus, bilateral angular gyri, left medial temporal lobe, and cingulate
gyrus. The largest cluster was found in the mPFC, along with two clusters in the left medial frontal
and superior frontal lobe (Table 2 & Figure 2a-b). When seeding in the mPFC there was, again,
significantly higher FC in ECPs from the seed to seven individual clusters including: within the
mPFC, bilateral insula, left medial frontal lobe, left angular gyrus, left superior frontal gyrus, and
right medial temporal lobe (Table 2 & Figure 2d-e).
In comparison, LCPs had higher FC to three of the 18 identified clusters that survived FWE correction
at p < 0.05. When seeding in the mPFC, clusters were found in the anterior cingulate cortex and right
superior frontal gyrus, whilst seeding in the PCC identified a cluster in the left angular gyrus (Table 2
& Figure 2c,f).
***INSERT TABLE 2***
***INSERT FIGURE 2***
Downloaded from https://academic.oup.com/sleep/advance-article-abstract/doi/10.1093/sleep/zsz033/5316210 by Ritsumeikan University user on 18 February 2019
Accepted Manuscript
10
Attentional Performance and Sleepiness
A significant interaction between circadian phenotype and time of day was found for PVT
performance (F(2, 72) = 4.9, p = 0.01) but not Stroop performance (F(2, 70) = 1.6, p = 0.22). The
main effect of time of day was significant for both PVT (F(2, 72) = 3.2, p = 0.048) and Stroop
performance (F(2, 70) = 3.8, p = 0.028) as well as the main effect of circadian phenotype for PVT
(F(1, 36) = 4.4, p = 0.044) but not Stroop (F(1, 35) = 3.7, p = 0.063) (Figure 3b,c). Post hoc tests
revealed that the source of group effect for PVT was the 08:00 h testing session, where LCPs’
performance was significantly worse than ECPs (p = 0.0058). Significant diurnal variations were
found for LCPs but not ECPs in both PVT and Stroop performance, showing that the source of time of
day effects were driven LCPs. LCPs morning PVT performance was significantly worse compared to
the afternoon and evening (p = 0.0079 and p = 0.0006). LCPs morning Stroop performance was
significantly better in the afternoon compared to morning (p = 0.035).
For the KSS, there was a significant interaction between time of day and circadian phenotype (F(2,72)
= 18.1, p < 0.0001), as well as a significant main effect of circadian phenotype (F(1,36) = 9.2, p =
0.0044) but not time of day (F(2,72) = 2.0, p = 0.15). Group effects were driven by LCPs being
significantly sleepier at 08:00 h compared to ECPs (p < 0.0001). The interaction effect revealed
significant diurnal variations in both groups with opposing relationships. ECPs were significantly
more sleepy in the evening (4.9 ± 0.4) compared to the morning (3.1 ± 0.4) (p = 0.0054). LCPs
showed the inverse relationship being significantly sleepier at 08:00 h (6.4 ± 0.3), compared to 14:00
h and 20:00 h (both p < 0.0001) (Figure 3a).
***INSERT FIGURE 3***
Predicting Attentional Performance and Sleepiness
Rs-FC could independently predict performance variables (Figure 4). Using FC values from regions
with higher FC in ECPs than LCPs, GEEs showed that rs-FC of the mPFC could predict PVT (W =
14.5, p < 0.0001) and Stroop performance (W = 9.0, p = 0.003). Rs-FC of the PCC (ECPs > LCPs)
could also predict PVT performance (W = 6.4, p = 0.012) but not Stroop performance (W = 2.5, p =
0.12). Sleepiness score could be predicted by rs-FC of the PCC (W = 6.0, p = 0.015) but not rs-FC of
the mPFC (W = 1.5, p = 0.22). No significant predictive effects of rs-FC were found for regions
higher in LCPs (LCPs > ECPs) for either seed.
Time of day was also a significant independent predictor of performance and sleepiness. Using the
mPFC model, time of day could predict PVT (W = 9.2, p = 0.01) but not Stroop performance (W =
5.1, p = 0.078). Using the PCC model, time of day was a significant predictor of both PVT and Stroop
Downloaded from https://academic.oup.com/sleep/advance-article-abstract/doi/10.1093/sleep/zsz033/5316210 by Ritsumeikan University user on 18 February 2019
Accepted Manuscript
11
performance (W = 6.3, p = 0.042 and W = 7.1, p = 0.028 respectively). Sleepiness could be
independently predicted by time of day (mPFC: W = 17.1, p < 0.0001 and PCC: W = 11.1, p = 0.004)
as well as by the interaction of rs-FC and time of day for both models (mPFC: W = 14.5, p = 0.001
and PCC: W = 8.7, p = 0.013).
In summary, averaged rs-FC of the mPFC from the regions higher in ECPs compared to LCPs
predicted better attentional performance i.e. faster reaction times in both PVT and Stroop
performance. Similarly, the equivalent measures from the PCC seed could predict better PVT
performance and lower daytime sleepiness but not Stroop performance. The interaction of time of day
and rs-FC predicted daytime sleepiness for both seeds. Time of day independently predicted
attentional performance and sleepiness variables in both models. Averaged rs-FC from regions
showing higher FC in LCPs compared to ECPs for both seeds showed no predictive effects on
attentional performance or sleepiness, with only time of day predicting PVT and Stroop performance.
***INSERT FIGURE 4***
Discussion
According to previous research only around 15% of the population falls into extreme or moderate
Early chronotypes (going to sleep from between 20:30 ? 23:00 h and waking between 04:30 ? 07:00
h),
7 meaning the majority of the population would not usually fit into the standard working schedule,
preferring to go to sleep and wake up later. Consequently, many individuals, in particular those with
extreme late preferences who can be classified as LCPs, are constantly fighting their innate circadian
phenotype and sleep patterns to fit into socio-professional routines.
Here we show, for the first time, fundamental differences in FC of the DMN between ECPs and LCPs
during a typical working day (08:00 h ? 20:00 h). Regardless of time of day, ECPs had higher rs-FC
than LCPs in the majority of regions identified. Many of the regions identified as having higher rs-FC
in ECPs are linked to cognitive function and control, including the right and left anterior insula (rAI
and lAI), two main regions which are also featured in the salience network. FC between the mPFC
and the rAI has previously been shown to correlate with cumulative habitual sleep duration,
22 and
with the current data this suggests that mPFC-insula FC during wakefulness could also be sensitive to
sleep timings and circadian phentoype. Given that connectivity between similar regions are associated
with either sleep duration or timing, these regions could be more broadly related to sleep and highlight
the potential importance of inter-network connectivity. Furthermore, rs-FC of these regions was
predictive of attentional performance measures i.e. reaction time and subjective sleepiness. While we
are not able to identify the causality of the relationships unambiguously within our experimental
design, this could suggest that the higher rs-FC of the DMN observed in ECPs over relatively
widespread regions mediates improved task performance. It is also important to note that whilst the
interpretation of FC can be partially based on activation studies using task-based fMRI, the
relationship between connectivity and activation is not straightforward and remains an active area of
research.
54, 55
Interactions between the brainstem arousal systems and ventrolateral preoptic nucleus of the
hypothalamus are known to play in determining circadian rhythmicity and sleep-wake cycles. The
impact of an underlying biological predisposition (e.g. circadian phenotype) to particular sleep-wake
patterns on brain function and subsequently behaviour has not previously been demonstrated, but is
Downloaded from https://academic.oup.com/sleep/advance-article-abstract/doi/10.1093/sleep/zsz033/5316210 by Ritsumeikan University user on 18 February 2019
Accepted Manuscript
12
consistent with previous observations linking FC to behavioural performance56 and habitual sleep
durations.
22 Therefore, an alternative proposal would be that there could be other brain regions, shown
here in DMN FC, that contribute to variability between circadian phenotypes. These differences in
intrinsic FC have not previously been linked to the known role of the DMN presenting an interesting
area for future research.
Of the 18 regions identified as being significantly different in terms of their FC between ECPs and
LCPs, the substantial majority (83%) demonstrated higher FC in ECPs. This suggests that an early
sleep-wake pattern is generally associated with higher FC from the primary nodes of the DMN. Since
the 08:00 h session required LCPs to wake earlier, these individuals were suffering from acute sleep
restriction. As a result, the morning session was expected to show the greatest difference between the
groups. PVT performance and sleepiness scores exhibited significant diurnal variations and were
significantly lower in LCPs compared to ECPs at 08:00 h, suggesting that these measures could be
sensitive to the curtailment in sleep. However, this result is not reflected in FC, which shows
consistent group differences at each time point but no significant diurnal variations. As such, these
findings could be due to more intrinsic circadian phenotype traits and not acute sleep restriction.
While LCPs tend to be heavily disrupted throughout their lifetimes when enforced to fit to
conventional societal days, those taking part in the current study were able to follow their own
preferred routines throughout the study and had comparable sleep parameters to ECPs (e.g. duration,
efficiency) with only sleep timings differing significantly. This would support the notion of LCPs
showing adverse effects when persistently following an earlier schedule during the work week, even
when trying to compensate on non-working ‘free’ days.
19 It is likely that a more chronic effect of
long-term misalignment, e.g. years of having to fit into school and subsequent work schedules, may
extend to impact on intrinsic brain properties even when individuals are able to follow their own
schedules for a period of two weeks. This is consistent with observations of continued cognitive
deficits following prolonged shift work, even after the shift work has ceased.
57 Therefore, these
findings may be underestimating the differences in FC and performance, which could be exacerbated
by acute disruption.
The increasingly sophisticated ability of fMRI to probe and quantify the human brain’s functional
architecture opens up new possibilities for understanding the impact of sleep and circadian
preferences at the level of the individual. While considerable advances have been made in
understanding the cellular and genetic underpinnings of sleep and circadian rhythmicity,
58 and
behavioural effects have been characterised,
7
only recently have the methods been available to study
their impacts on the human brain in vivo. These developments are crucial, given the intrinsic
importance of understanding human brain function and the commonly-held view that the primary
purpose of sleep is for the brain.
59 The use of rs-FC is particularly attractive for this endeavour
because of the pervasiveness of the behavioural and cognitive effects of sleep patterns and circadian
phenotype, which lend themselves to characterisation of intrinsic network function rather than the
more limited task responses. More broadly, the approach we have taken provides important
information about how intrinsic lifestyle factors and biological phenotypes are reflected in the brain’s
default state (DMN), suggesting new avenues for understanding individual differences in behaviour.
Our analysis revealed that rs-FC of the DMN can independently predict measures of task performance
and subjective daytime sleepiness. This suggests that the higher strength of rs-FC between these
regions, the better an individual performs in an attention task and the less sleepy they feel. Since our
analysis used seeds within the DMN, one could infer that the functional integrity of connections from
key regions of the DMN facilitates attentional performance, and that perturbations of the DMN
associated with misalignment are detrimental (caveats regarding causality as discussed above
Downloaded from https://academic.oup.com/sleep/advance-article-abstract/doi/10.1093/sleep/zsz033/5316210 by Ritsumeikan University user on 18 February 2019
Accepted Manuscript
13
notwithstanding). The DMN is important in maintenance of consciousness, and includes cognitive
domains sub-served by the frontal cortex.
60 Altered functional connectivity of the DMN has been
reported in a number of psychiatric disorders, suggesting that disrupted integrity of this network is
linked to psychological processes (see 61 for review). Although decreased FC does not always relate to
decreased task performance, reduced connectivity from mPFC and PCC regions of the DMN has been
proposed to underlie impairments in attentional control, working memory and emotional processing.
61
The majority of this research, investigating both DMN connectivity and activation, has reported
decreased FC in disorders such as Alzheimer’s, attention deficit hyperactivity disorder and autism.
Conversely, an increase in FC from the subgenual anterior cingulate has been associate with
depression.
62 We find that ECPs have higher rs-FC from the majority of significant clusters. However,
of the three clusters that we identify as having higher rs-FC in LCPs, one was in the anterior cingulate
cortex. Since LCPs are a group who have frequently been linked to higher rates of depression, this
result has potentially uncovered an interesting avenue for future work and highlights that interpreting
increases/decreases in rs-FC are not always straightforward. Adding to the growing body of research
into the consequences of disrupted DMN rs-FC, we now show that circadian and sleep variations can
contribute to understanding how the integrity of the DMN at rest could hold a key role in achieving
optimal cognitive functioning (shown here using attentional tasks).
Previous research has shown diurnal variations in FC of resting state networks, suggesting that
different ICNs have varying sensitivity to time of day.
23, 37 However, although in the current study
diurnal variations were found in attentional performance and sleepiness measures, using a flexible
factorial design to account for the complex study design, we found that the effect of circadian
phenotype on rs-FC was much more marked than the effect of time of day. This suggests that rs-FC of
the DMN is primarily sensitive to stable, trait-like differences between the two groups rather than
more dynamic state-like effects. This is consistent with the fact that habitual sleep patterns have been
linked with anatomical63 as well as functional22 differences, suggesting long term modifications to
brain function can occur as a result of modifications to the underlying structure. However, it is
possible that the examination of additional networks beyond the DMN and the use of dynamic FC64
would identify state-like impacts of circadian misalignment which might be more sensitive to the
effects of time of day. It is also important to note that these data were gathered during typical working
hours (08:00 h ? 20:00 h) which could have resulted in failure to record time points in which LCPs
could have shown higher FC and better attentional performance. However, LCPs are under constant
pressure to fight again their endogenously driven circadian rhythms to fit into socio-professional
imposed schedules. This could cause them to be in a state of ‘perpetual chronodisruption’ despite
being able to follow their preferred schedules for the duration of this study.
There are a number of limitations to this study. Firstly, to be able to investigate how ECPs and LCPs
behave during a ‘normal socially constrained day’ e.g. 08:00 h to 20:00 h, the study was designed
using clock time instead of scheduling testing based on internal biological time. Although this design
does not allow sleep and circadian influences to be separated, there is increasing need to carry out
‘real world’ studies to increase external validity as behaviour is impacted by both factors. In addition,
we only investigated one ICN, the DMN, and therefore limit the ability to explore more complex
whole brain inter- and intra- network functional connectivity. Both the mPFC and PCC regions of the
DMN were used as seeds because although the DMN is a coherent network, each of the regions that
comprise it also have other functions and potentially have different susceptibility to the impact of
circadian phenotype and time of day. Since the DMN is the most widely studied ICN, holds a key
role in maintenance of consciousness, is affected by sleep, and disruption of this network has been
linked to impaired attentional control, there was a strong rationale to choose it as the network of
interest and provides a useful starting point for a relatively unexplored field. Nonetheless, studying
Downloaded from https://academic.oup.com/sleep/advance-article-abstract/doi/10.1093/sleep/zsz033/5316210 by Ritsumeikan University user on 18 February 2019
Accepted Manuscript
14
the impact of circadian phenotype on other ICNs, as well as other measures of cognition which could
be impacted differently, would be an important next step for future work. Similarly, given that ECPs
and LCPs differ significantly in their physiology, another important step would be to explore
biological and genetic mechanisms behind the observed changes in rs-FC.
The majority of variables were evenly matched between the groups with the exception of sleep
timings (onset/offset) and circadian phase markers (DLMO). Sleep efficiency values were relatively
low for healthy controls, although sleep durations are in the normal range for this cohort of young
adults and additional analysis showed no significant correlations of sleep efficiency and rs-FC. This
suggests that there is no baseline sleep debt effect and both groups are not acutely suffering from
sleep debt during the course of this study. This allows us to confidently state we have distinct
circadian phenotype differences. We did have a slight but significant difference in age between the
groups, although not sufficient to account for the differences since studies examining the relationship
between FC of the DMN and age demonstrate that FC is stable from young adulthood until 50-60y.
65
Throughout the duration of the study, participants were following their preferred routines to allow a
true indication of the impact of circadian phenotype in the absence of masking effects. However, this
is likely to underestimate the practical impact on LCPs of conforming to a societal day, since in
reality the LCPs are likely to have an additional burden of sleep debt which will have its own negative
effect. In our study, the 08:00 h session will have caused the LCPs to wake earlier than usual and,
therefore, be affected by sleep restriction. Although we are not able to determine the extent of
shortening the sleep period before the morning session, the lack of diurnal variations found in FC
suggests that we have identified more circadian trait-like differences between the groups. In addition,
since LCPs commonly have to get up prior to habitual wake up time, this study was specifically
conducted to investigate these individuals in a ‘real-world’ situation. Dissociating the impact of
circadian misalignment and sleep deprivation is often difficult, with protocols such as forced
desynchrony and constant routine generally providing the gold standard. However, these protocols
have disadvantages in terms of their ability to understand the impact of differences in habitual sleep
patterns and circadian phenotype on the brain and behaviour. Future work will need to make use of
these protocols and to study individuals who are acutely misaligned in order to explore the longer
term effect on the brain of chronic misalignment.
Conclusions
In summary, we find that there are fundamental differences in the intrinsic FC of the DMN between
ECPs and LCPs during a typical ‘societally constrained’ working day. Rs-FC of the DMN can predict
attentional performance measures and subjective sleepiness differences, which are also modulated by
time of day. These findings could contribute to the neural basis underlying performance and health
differences between ECPs and LCPs in the real world and have implications for future research.
Firstly, an individual’s circadian phenotype should be a factor that is taken into account when using
fMRI for research and clinical applications, as should habitual sleep status and duration.
22 Secondly,
we provide a deeper understanding of the biological basis of individual differences in the DMN that
may be associated with negative outcomes in LCPs. Finally, LCPs are impaired during typical
socially constrained days, which could result in lower FC and lead to their diminished morning
performance and increased daytime sleepiness. This suggests a need to be more conscious about how
to manage time on an individual basis in order to maximise productivity and minimise health risks.
Downloaded from https://academic.oup.com/sleep/advance-article-abstract/doi/10.1093/sleep/zsz033/5316210 by Ritsumeikan University user on 18 February 2019
Accepted Manuscript
15
Abbreviations list
ECP: Early circadian phenotype
LCP: Late circadian phenotype
ICNs: Intrinsically connected networks
FC: Functional connectivity
Rs-FC: Resting-state functional connectivity
DMN: Default mode network
MSFsc: Corrected mid-sleep on free days
DLMO: Dim light melatonin onset
KSS: Karolinska Sleepiness Scale
PVT: Psychomotor vigilance task
Acknowledgements
This work was supported by funding from the Biotechnology and Biological Sciences Research
Council (BBSRC, BB/J014532/1) and the Engineering and Physical Sciences Research Council
(EPSRC, EP/J002909/1) as well as the Brazilian Institute of Neuroscience and Neurotechnology
(FAPESP #2013/07559-3) and Birmingham University Imaging Centre (BUIC). E.F.C was supported
by a Wellcome Trust Institutional Strategic Support Fund (ISSF) Scheme accelerator fellowship
(Wellcome 204846/Z/16/Z). Our sincere thanks are to all participants, Stockgrand Ltd for assay
reagents and Drs Joseph Galea, Bernhard Staresina and Stephane de Brito for invaluable comments on
earlier versions of the manuscript.
Author Contributions
E.F.C. and A.P.B. designed the study with contributions from D.J.S. B.M.C. developed the
neuroimaging software used for the analyses and were involved in analysis of the data. E.F.C
collected and processed the MRI data with contributions from A.P.B. and B.M.C. RIA analyses was
performed by B.M. E.F.C wrote the manuscript with contributions from A.P.B. All other authors
commented on the manuscript.
Disclosure Statement
B.M. and D.J.S. are co-directors of Stockgrand Ltd. The authors declare no other competing financial
interests. Non-financial disclosure: none.
Downloaded from https://academic.oup.com/sleep/advance-article-abstract/doi/10.1093/sleep/zsz033/5316210 by Ritsumeikan University user on 18 February 2019
Accepted Manuscript
16
Reference list
1. Altevogt BM, Colten HR. Sleep disorders and sleep deprivation: an unmet public health
problem: National Academies Press, 2006.
2. Devore EE, Grodstein F, Schernhammer ES. Shift Work and Cognition in the Nurses Health
Study. Am J Epidemiol 2013;178:1296-300.
3. Rajaratnam SM, Arendt J. Health in a 24-h society. The Lancet 2001;358:999-1005.
4. Savvidis C, Koutsilieris M. Circadian rhythm disruption in cancer biology. Mol Med
2012;18:1249-60.
5. Lange T, Dimitrov S, Born J. Effects of sleep and circadian rhythm on the human immune
system. Annals of the New York Academy of Sciences 2010;1193:48-59.
6. Banks S, Dinges DF. Behavioral and physiological consequences of sleep restriction. J Clin
Sleep Med 2007;3:519-28.
7. Roenneberg T, Kuehnle T, Juda M, et al. Epidemiology of the human circadian clock. Sleep
Med Rev 2007;11:429-38.
8. Horne JA, Ostberg O. Individual differences in human circadian rhythms. Biological
Psychology 1977;5:179-90.
9. Taillard J, Philip P, Coste O, Sagaspe P, Bioulac B. The circadian and homeostatic
modulation of sleep pressure during wakefulness differs between morning and evening chronotypes.
Journal of sleep research 2003;12:275-82.
10. Kanerva N, Kronholm E, Partonen T, et al. Tendency toward eveningness is associated with
unhealthy dietary habits. Chronobiology international 2012;29:920-7.
11. Ross KM, Graham Thomas J, Wing RR. Successful weight loss maintenance associated with
morning chronotype and better sleep quality. J Behav Med 2016;39:465-71.
12. Lastella M, Roach GD, Halson SL, Sargent C. The Chronotype of Elite Athletes. Journal of
human kinetics 2016;54:219-25.
13. Owens JA, Dearth-Wesley T, Lewin D, Gioia G, Whitaker RC. Self-regulation and sleep
duration, sleepiness, and chronotype in adolescents. Pediatrics 2016;138:e20161406.
14. Wittmann M, Paulus MP, Roenneberg T. Decreased psychological well-being in late
‘chronotypes’ is mediated by smoking and alcohol consumption. Subst Use Misuse 2010;45:15-30.
15. Merikanto I, Lahti T, Kronholm E, et al. Evening types are prone to depression.
Chronobiology international 2013;30:719-25.
16. Alvaro PK, Roberts RM, Harris JK. The independent relationships between insomnia,
depression, subtypes of anxiety, and chronotype during adolescence. Sleep medicine 2014;15:934-41.
17. Roenneberg T, Allebrandt KV, Merrow M, Vetter C. Social Jetlag and Obesity. Current
Biology 2012;22:939-43.
18. Knutson KL, von Schantz M. Associations between chronotype, morbidity and mortality in
the UK Biobank cohort. Chronobiology international 2018:1-9.
19. Wittmann M, Dinich J, Merrow M, Roenneberg T. Social jetlag: misalignment of biological
and social time. Chronobiol Int 2006;23:497-509.
20. Horovitz SG, Braun AR, Carr WS, et al. Decoupling of the brain's default mode network
during deep sleep. Proceedings of the National Academy of Sciences 2009;106:11376-81.
Downloaded from https://academic.oup.com/sleep/advance-article-abstract/doi/10.1093/sleep/zsz033/5316210 by Ritsumeikan University user on 18 February 2019
Accepted Manuscript
17
21. De Havas JA, Parimal S, Soon CS, Chee MW. Sleep deprivation reduces default mode
network connectivity and anti-correlation during rest and task performance. Neuroimage
2012;59:1745-51.
22. Khalsa S, Mayhew SD, Przezdzik I, et al. Variability in cumulative habitual sleep duration
predicts waking functional connectivity. Sleep 2016;39:87.
23. Blautzik J, Vetter C, Peres I, et al. Classifying fMRI-derived resting-state connectivity
patterns according to their daily rhythmicity. NeuroImage 2013;71:298-306.
24. Gusnard DA, Raichle ME. Searching for a baseline: functional imaging and the resting human
brain. Nature Reviews Neuroscience 2001;2:685-94.
25. Raichle ME, MacLeod AM, Snyder AZ, Powers WJ, Gusnard DA, Shulman GL. A default
mode of brain function. Proceedings of the National Academy of Sciences 2001;98:676-82.
26. Danielson NB, Guo JN, Blumenfeld H. The default mode network and altered consciousness
in epilepsy. Behav Neurol 2011;24:55-65.
27. Leech R, Sharp DJ. The role of the posterior cingulate cortex in cognition and disease. Brain :
a journal of neurology 2013;137:12-32.
28. Gui D, Xu S, Zhu S, et al. Resting spontaneous activity in the default mode network predicts
performance decline during prolonged attention workload. NeuroImage 2015;120:323-30.
29. Esposito F, Aragri A, Latorre V, et al. Does the default-mode functional connectivity of the
brain correlate with working-memory performances? Arch Ital Biol. 2009;147:11-20.
30. Mohan A, Roberto AJ, Mohan A, et al. Focus: the aging brain: the significance of the default
mode network (DMN) in neurological and neuropsychiatric disorders: a review. The Yale journal of
biology and medicine 2016;89:49.
31. Greicius MD, Srivastava G, Reiss AL, Menon V. Default-mode network activity distinguishes
Alzheimer's disease from healthy aging: evidence from functional MRI. Proceedings of the National
Academy of Sciences 2004;101:4637-42.
32. Sheline YI, Barch DM, Price JL, et al. The default mode network and self-referential
processes in depression. Proceedings of the National Academy of Sciences 2009;106:1942-7.
33. Song M, Zhou Y, Li J, et al. Brain spontaneous functional connectivity and intelligence.
Neuroimage 2008;41:1168-76.
34. Van Den Heuvel MP, Stam CJ, Kahn RS, Pol HEH. Efficiency of functional brain networks
and intellectual performance. Journal of Neuroscience 2009;29:7619-24.
35. Marek T, Fafrowicz M, Golonka K, et al. Diurnal patterns of activity of the orienting and
executive attention neuronal networks in subjects performing a Stroop-like task: a functional magnetic
resonance imaging study. Chronobiol Int 2010;27:945-58.
36. Matchock RL, Mordkoff JT. Chronotype and time-of-day influences on the alerting,
orienting, and executive components of attention. Exp Brain Res 2009;192:189-98.
37. Hodkinson DJ, O'daly O, Zunszain PA, et al. Circadian and homeostatic modulation of
functional connectivity and regional cerebral blood flow in humans under normal entrained
conditions. Journal of Cerebral Blood Flow & Metabolism 2014;34:1493-9.
38. Roenneberg T, Wirz-Justice A, Merrow M. Life between clocks: daily temporal patterns of
human chronotypes. J Biol Rhythms 2003;18:80-90.
39. Moreno C, Vasconcelos S, Marqueze E, et al. Sleep patterns in Amazon rubber tappers with
and without electric light at home. Sci Rep-Uk 2015;5:14074.
40. Berry RB, Brooks R, Gamaldo CE, Harding SM, Marcus C, Vaughn B. The AASM manual
for the scoring of sleep and associated events. Rules, Terminology and Technical Specifications,
Darien, Illinois, American Academy of Sleep Medicine 2012.
41. de Campos BM, Coan AC, Lin Yasuda C, Casseb RF, Cendes F. Large-scale brain networks
are distinctly affected in right and left mesial temporal lobe epilepsy. Hum Brain Mapp 2016;37:3137-
52.
42. Kasper L, Bollmann S, Diaconescu AO, et al. The PhysIO toolbox for modeling physiological
noise in fMRI data. Journal of Neuroscience Methods 2017;276:56-72.
43. Penny WD, Friston KJ, Ashburner JT, Kiebel SJ, Nichols TE. Statistical parametric mapping:
the analysis of functional brain images: Academic press, 2011.
Downloaded from https://academic.oup.com/sleep/advance-article-abstract/doi/10.1093/sleep/zsz033/5316210 by Ritsumeikan University user on 18 February 2019
Accepted Manuscript
18
44. Power JD, Barnes KA, Snyder AZ, Schlaggar BL, Petersen SE. Spurious but systematic
correlations in functional connectivity MRI networks arise from subject motion. Neuroimage
2012;59:2142-54.
45. Power JD, Mitra A, Laumann TO, Snyder AZ, Schlaggar BL, Petersen SE. Methods to detect,
characterize, and remove motion artifact in resting state fMRI. Neuroimage 2014;84.
46. Shirer WR, Ryali S, Rykhlevskaia E, Menon V, Greicius MD. Decoding Subject-Driven
Cognitive States with Whole-Brain Connectivity Patterns. Cereb Cortex 2012;22:158-65.
47. Dinges DF, Powell JW. Microcomputer analyses of performance on a portable, simple visual
RT task during sustained operations. Behavior Research Methods, Instruments, & Computers
1985;17:652-5.
48. Stroop JR. Studies of interference in serial verbal reactions. J Exp Psychol 1935;18:643-62.
49. Akerstedt T, Gillberg M. Subjective and objective sleepiness in the active individual. Int J
Neurosci 1990;52:29-37.
50. Benjamini Y, Hochberg Y. Controlling the false discovery rate: a practical and powerful
approach to multiple testing. Journal of the royal statistical society. Series B (Methodological)
1995:289-300.
51. Hasler G, Buysse DJ, Klaghofer R, et al. The association between short sleep duration and
obesity in young adults: a 13-year prospective study. Sleep 2004;27:661-6.
52. Lauderdale DS, Knutson KL, Yan LL, Liu K, Rathouz PJ. Self-reported and measured sleep
duration: how similar are they? Epidemiology (Cambridge, Mass.) 2008;19:838-45.
53. Ramgopal S, Vendrame M, Shah A, et al. Circadian patterns of generalized tonic?clonic
evolutions in pediatric epilepsy patients. Seizure 2012;21:535-9.
54. Gonzalez-Castillo J, Bandettini PA. Task-based dynamic functional connectivity: Recent
findings and open questions. Neuroimage 2018;180:526-33.
55. Tavor I, Parker Jones O, Mars RB, Smith SM, Behrens TE, Jbabdi S. Task-free MRI predicts
individual differences in brain activity during task performance. Science 2016;352:216-20.
56. Rosenberg MD, Finn ES, Scheinost D, et al. A neuromarker of sustained attention from
whole-brain functional connectivity. Nature Neuroscience 2016;19:165-71.
57. Marquie JC, Tucker P, Folkard S, Gentil C, Ansiau D. Chronic effects of shift work on
cognition: findings from the VISAT longitudinal study. Occup Environ Med 2015;72:258-64.
58. Lane JM, Vlasac I, Anderson SG, et al. Genome-wide association analysis identifies novel
loci for chronotype in 100,420 individuals from the UK Biobank. Nat Commun 2016;7:10889.
59. Hobson JA. Sleep is of the brain, by the brain and for the brain. Nature 2005;437:1254.
60. Greicius MD, Krasnow B, Reiss AL, Menon V. Functional connectivity in the resting brain: a
network analysis of the default mode hypothesis. Proc Natl Acad Sci U S A 2003;100:253-8.
61. Broyd SJ, Demanuele C, Debener S, Helps SK, James CJ, Sonuga-Barke EJ. Default-mode
brain dysfunction in mental disorders: a systematic review. Neurosci Biobehav Rev 2009;33:279-96.
62. Connolly CG, Wu J, Ho TC, et al. Resting-State Functional Connectivity of Subgenual
Anterior Cingulate Cortex in Depressed Adolescents. Biol Psychiat 2013;74:898-907.
63. Khalsa S, Hale JR, Goldstone A, et al. Habitual sleep durations and subjective sleep quality
predict white matter differences in the human brain. Neurobiology of Sleep and Circadian Rhythms
2017;3:17-25.
64. Beaty RE, Chen Q, Christensen AP, Qiu J, Silvia PJ, Schacter DL. Brain networks of the
imaginative mind: Dynamic functional connectivity of default and cognitive control networks relates
to openness to experience. Human brain mapping 2018;39:811-21.
65. Andrews-Hanna JR, Snyder AZ, Vincent JL, et al. Disruption of large-scale brain systems in
advanced aging. Neuron 2007;56:924-35.
Downloaded from https://academic.oup.com/sleep/advance-article-abstract/doi/10.1093/sleep/zsz033/5316210 by Ritsumeikan University user on 18 February 2019
Accepted Manuscript
19
Table 1. Summary of demographic, actigraphic and physiological variables for Early
(ECPs) and Late (LCPs) circadian phenotypes. Values are shown as mean ± SEM unless
specified. Significance is shown with a
parametric tests, b
non-parametric tests or c
Fisher’s
exact test. Phase angle is calculated by the interval time between dim light melatonin onset
and sleep onset.
Variable Measured (mean ± SEM) ECPs LCPs Significance
Demographic variables
Sample Size N = 16 N = 22 n/a
Number of Scans/Testing Sessions N = 48 N = 66 n/a
Percentage of Males/Females (%) M = 43.8 M = 31.8 ns
c
F = 56.3 F = 68.2 nsc
Age (years) (mean ± s.d.) 24.7 ± 4.0 21.2 ± 3.3 p = 0.028a
Height (cm) 171.3 ± 2.0 171.1 ± 2.4 nsa
Weight (kg) 66.4 ± 2.8 67.1 ± 2.1 ns
a
MCTQ Score (hh:mm) 02:24 ± 00:10 06:52 ± 00:17 p < 0.0001a
Actigraphic variables
Sleep Onset (hh:mm) 22:57 ± 00:10 02:27 ± 00:19 p < 0.0001a
Wake Up Time (hh:mm) 06:33 ± 0.10 10:13 ± 00:18 p < 0.0001a
Sleep Duration (h) 7.59 ± 0.18 7.70 ± 0.14 nsa
Sleep Efficiency (%) 79.29 ± 1.96 77.23 ± 1.14 nsa
Sleep Onset Latency (hh:mm) 00:25 ± 00:06 00:25 ± 00:03 nsb
Physiological variables
Phase Angle (hh:mm) 02:28 ± 00:16 02:34 ± 00:18 nsa
Dim Light Melatonin Onset (hh:mm) 20:27 ± 00:16 23:55 ± 00:26 p < 0.0001a
Cortisol Peak Time (hh:mm) 07:04 ± 00:16 11:13 ± 00:23 p < 0.0001a
External variables (between sessions)
Downloaded from https://academic.oup.com/sleep/advance-article-abstract/doi/10.1093/sleep/zsz033/5316210 by Ritsumeikan University user on 18 February 2019
Accepted Manuscript
20
Table 2. Summary of significant brain regions (FWE, p < 0.05) between Early circadian phenotypes
(ECPs) and Late circadian phenotypes (LCPs) when seeding in the posterior cingulate cortex (PCC)
and medial prefrontal cortex (mPFC).
Region Contrast Seed
regio
n
Cluster
size
(voxels)
MNI centroid
coordinates
[x y z]
Maximu
m t-score
Medial Prefrontal Cortex ECPs > LCPs PCC 789 [-2 72 12] 13.71
Right Angular Gyrus ECPs > LCPs PCC 481 [46 -68 26] 8.14
Precuneus ECPs > LCPs PCC 431 [0 -64 18] 9.73
Left Angular Gyrus ECPs > LCPs PCC 257 [-54 -62 18] 14.75
Left Medial Temporal
Lobe
ECPs > LCPs PCC 237 [-58 -6 -24] 7.94
Left Superior Frontal
Gyrus
ECPs > LCPs PCC 212 [-18 60 26] 7.91
Left Medial Frontal Lobe ECPs > LCPs PCC 173 [-46 16 56] 8.71
Cingulate Gyrus ECPs > LCPs PCC 150 [-16 -42 26] 18.90
Left Angular Gyrus LCPs > ECPs PCC 428 [-32 -54 26] 16.29
Medial Prefrontal Cortex ECPs > LCPs mPFC 384 [2 70 6] 10.99
Left Anterior Insula ECPs > LCPs mPFC 378 [-26 14 -24] 8.87
Right Anterior Insula ECPs > LCPs mPFC 241 [26 18 -20] 9.36
Left Medial Frontal Lobe ECPs > LCPs mPFC 160 [-44 16 56] 9.62
Left Angular Gyrus ECPs > LCPs mPFC 134 [-56 -58 18] 10.19
Left Superior Frontal
Gyrus
ECPs > LCPs mPFC 111 [-4 68 28] 8.96
Right Medial Temporal
Lobe
ECPs > LCPs mPFC 108 [68 -12 -8] 6.15
Anterior Cingulate LCPs > ECPs mPFC 233 [22 44 10] 7.20
Right Superior Frontal
Gyrus
LCPs > ECPs mPFC 161 [22 42 52] 6.55
Hours since last meal (h) 3.58 ± 0.55 5.07 ± 0.58 nsb
Hours since caffeine (h) 8.47 ± 0.67 7.85 ± 0.82 nsb
Hours since exercise (h) 6.78 ± 0.74 7.44 ± 0.74 nsb
Hours since natural light exposure (h) 5.87 ± 0.80 3.51 ± 0.58 nsb
Hours since indoor light exposure (h) 1.88 ± 0.38 3.32 ± 0.51 nsb
Downloaded from https://academic.oup.com/sleep/advance-article-abstract/doi/10.1093/sleep/zsz033/5316210 by Ritsumeikan University user on 18 February 2019
Accepted Manuscript
21
Figure Captions
Figure 1. Linear relationships between corrected mid-sleep on free days (MSFsc) and biological
phase markers to validate circadian phenotyping. a) Dim light melatonin onset, b) Sleep onset, c)
Time of peak cortisol concentration, d) Wake up time. MSFsc is displayed as time of day (h) on the x
axis. Statistical analysis was carried out using linear regression analysis. Significance (**** = p <
0.0001) and R2
values are shown in the bottom right corner.
Figure 2. Resting state functional connectivity (rs-FC) of the Default Mode Network between
Early and Late circadian phenotypes (ECP/LCP). Z-transformed connectivity maps show
significant clusters (FWE corrected p < 0.05 at voxel level and subsequent cluster level) and t-score
scales for each contrast are shown in the center. Overall results from each seed are shown in a/d with
results from each time point (hours) represented in b/c and e/f. a) Summary results from the posterior
cingulate cortex (PCC) seed with diurnal variations between circadian phenotype groups plotted in b)
and c). d) Summary results from medial prefrontal cortex seed (mPFC) with diurnal variations
between circadian phenotype groups plotted in e) and f). Significant regions at the whole group level
are represented in grayscale. Regions higher in ECPs (ECPs > LCPs) are shown in red and regions
higher in LCPs (LCPs > ECPs) in green. Statistical analysis for a) and d) was carried out using a
flexible factorial model in SPM12. Two-way ANOVA was used to analyse group and time of day
differences in b), c), e) and f). * = p < 0.05, *** = p < 0.001, **** = p < 0.0001.
Figure 3. Nonlinear regression curves to show diurnal variations in sleepiness, Psychomotor
vigilance (PVT) and Stroop performance. a) Subjective sleepiness score measured with the
Karolinska Sleepiness Scale. b) PVT performance (reaction time in seconds), c) Stroop performance
(reaction time in seconds) for Early circadian phenotypes (white) and Late circadian phenotypes
(grey). Clock time of test (h) is shown on the x axis for each parameter. Statistical analysis was
carried out using two-way ANOVA. Post hoc multiple comparison tests were run to determine group
and time of day effects. * = p < 0.05, ** = p < 0.01, *** = p < 0.001, **** = p < 0.0001.
Downloaded from https://academic.oup.com/sleep/advance-article-abstract/doi/10.1093/sleep/zsz033/5316210 by Ritsumeikan University user on 18 February 2019
Accepted Manuscript
22
Figure 4. Summary of predictive analysis using resting state functional connectivity (rsFC) to predict attentional performance and subjective daytime sleepiness (black boxes).
Solid arrows indicate the predictive effects of rs-FC on attentional performance (psychomotor
vigilance task, PVT and Stroop task) and sleepiness variables for models using data from
seeds in the medial prefrontal (mPFC) and posterior cingulate (PCC) cortices. Dotted lines
and red boxes indicate where time of day or the interaction of time of day and rs-FC was also
found to be a significant factor.
Downloaded from https://academic.oup.com/sleep/advance-article-abstract/doi/10.1093/sleep/zsz033/5316210 by Ritsumeikan University user on 18 February 2019
Accepted Manuscript
23
Figure 1
Downloaded from https://academic.oup.com/sleep/advance-article-abstract/doi/10.1093/sleep/zsz033/5316210 by Ritsumeikan University user on 18 February 2019
Accepted Manuscript
24
Figure 2
Downloaded from https://academic.oup.com/sleep/advance-article-abstract/doi/10.1093/sleep/zsz033/5316210 by Ritsumeikan University user on 18 February 2019
Accepted Manuscript
25
Figure 3
Downloaded from https://academic.oup.com/sleep/advance-article-abstract/doi/10.1093/sleep/zsz033/5316210 by Ritsumeikan University user on 18 February 2019
Accepted Manuscript
26
Figure 4
Downloaded from https://academic.oup.com/sleep/advance-article-abstract/doi/10.1093/sleep/zsz033/5316210 by Ritsumeikan University user on 18 February 2019
▲上へ ★阿修羅♪ > 不安と不健康18掲示板 次へ 前へ
投稿コメント全ログ コメント即時配信 スレ建て依頼 削除コメント確認方法
▲上へ ★阿修羅♪ > 不安と不健康18掲示板 次へ 前へ

すべてのページの引用、転載、リンクを許可します。確認メールは不要です。引用元リンクを表示してください。