http://www.asyura2.com/17/jisin22/msg/776.html
Tweet |
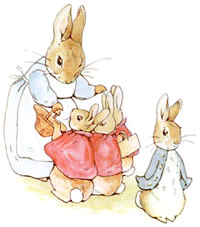
米 温暖化シミュレーション 被害地域の人口は想定の3倍か
2019年10月30日 5時03分
気候変動による海面の上昇によって影響を受ける人の数は、これまでの想定の3倍に上る可能性があることが、アメリカの団体のシミュレーションで明らかになり、専門家は「私たちが生きているうちに、都市や経済のありかたに大きな影響を与える可能性がある」と警告しています。
今月29日付けのイギリスの科学雑誌「ネイチャー・コミュニケーションズ」に掲載された論文によりますと、気候変動の研究者が参加するアメリカのNPOはAIの開発などに使われる「機械学習」を応用してこれまでのシミュレーションの精度を高める新たな手法を開発しました。
この手法を使って世界135か国のデータに基づき、気候変動に伴う海面上昇の影響を再検討したところ、2050年までに水没するなどの影響を受ける可能性がある海沿いの地域の人口は、古いシミュレーションモデルによる想定のおよそ3倍になったということです。
中でも、アジア地域での影響が大きく、仮に温室効果ガスの排出量がおおよそパリ協定で目標としたレベルに削減されたとしても、現在、2億3700万人が住む地域が定期的に洪水に見舞われるとしています。
このうち、中国ではこれまでの想定の3.2倍にあたる9300万人、バングラデシュでは8.4倍の4200万人、インドでは7.2倍の3600万人が影響を受けるとしています。
また、日本はこれまでのシミュレーションより70万人多い530万人が影響を受けるとしています。
論文の著者のスコット・カルプ博士は「私たちが生きているうちに、気候変動が都市や経済のありかたに大きな影響を与える可能性がある。各国は、住民を守るため、沿岸部の水害対策を絶えず迫られることになる」と指摘しています。
あわせて読みたい
アフリカ南部でゾウが大量死 厳しい干ばつで餓死
2019年10月23日 20時02分
厳しい干ばつが続くアフリカ南部のジンバブエとボツワナで、先月から今月にかけて合わせて150頭以上のゾウが死んでいるのが相次いで見つかり、地球温暖化による生態系への影響が懸念されています。
アフリカ南部のジンバブエの国立公園で、先月から今月にかけて、少なくとも55頭のゾウが死んでいるのが見つかりました。
国立公園が調査した結果、厳しい干ばつによる食料や水不足で、ゾウが餓死したとしていて、国立公園の担当者は現地メディアの取材に対し「これまでの状況の中でも極めて悪く、生きているゾウも食料不足に苦しんでいる。乾季に入れば状況はますます悪くなるだろう」と話しています。
また隣接するボツワナの政府はこの2か月で国立公園で生息していたゾウおよそ100頭が死んだことを明らかにしました。
政府はゾウが長時間にわたって食料を探し続けて餓死したことや、草が枯れて土まで食べてしまい、土の中の細菌に感染した可能性があるとしています。
環境保護に取り組む国際NGO、WWFジャパンによりますと、アフリカ南部にはおよそ29万頭のアフリカゾウが生息しているものの、象牙の密猟や違法な取り引きで個体数が減り続けているということです。
WWFジャパンは「地球温暖化によって引き起こされる干ばつで生息地の環境が変わり、ゾウの個体数がさらに減る可能性がある」として懸念を表明しています。
地球温暖化考慮の水害対策に転換へ 国交省
2019年10月19日 4時06分
今回の台風19号による豪雨など、地球温暖化の影響で水害の規模が大きくなることが懸念されています。こうした中、国土交通省は、これまで過去の最大規模の水害を基準に行っていた対策から、温暖化による気温上昇を考慮した対策に転換していくことになりました。
国土交通省は、去年4月に気象や河川の専門家などでつくる検討会を設置し、地球温暖化を考慮した水害対策について話し合っていて、18日、提言が発表されました。
それによりますと、地球温暖化によって気温が2度上昇すると、100年から200年に一度の豪雨の発生頻度がおよそ2倍になるという気象庁などの予測をもとに、これまで過去の最大規模の水害を基準に行っていた対策から、地球温暖化による気温上昇を考慮した対策に転換していく必要があると提言しています。
これを受けて国土交通省は、今回、決壊した堤防があるところを含めた全国の河川について、堤防やダムなどの施設をどう強化していくか検討し、それぞれの整備計画に反映させていくことにしています。
「温暖化を否定する者に隠れ家ない」国連事務総長が意見広告
2019年10月4日 10時23分
国連のグテーレス事務総長は、地球温暖化をめぐって、世界中の新聞や雑誌などに意見広告を出し「地球温暖化を否定する者に隠れ家(が)はない」と強いことばを使って、対策に消極的な国に働きかけを続ける考えを示しました。
国連のグテーレス事務総長は3日、地球温暖化をめぐって、イギリスの新聞「ガーディアン」やフランスの「ル・モンド」、それにアメリカの時事週刊誌「ザ・ネイション」といった世界250以上の新聞や雑誌などに意見広告を出しました。
この中でグテーレス事務総長は、先月、国連総会で開いた地球温暖化対策サミットで、70以上の国や100以上の自治体が表明した2050年までに温室効果ガスを実質ゼロにする約束について、ことし12月に南米チリで開く地球温暖化の会議「COP25」で、各国から実行に移すための説明を求めるとしています。
そのうえで「あまりに多くの国が石炭火力にとりつかれている。地球温暖化を否定する者に隠れ家はない」と強いことばを使って、対策に消極的な国に働きかけを続ける考えを示しました。
最後にグテーレス事務総長は「多くの若者や市民、企業など幅広い分野の人たちが立ち上がっているが、さらに多くの人たちの行動が必要で道のりは遠い」として、温暖化対策への支持と行動を呼びかけています。
関連・注目ワード
https://www3.nhk.or.jp/news/html/20191030/k10012156171000.html
海面上昇のリスク人口は従来推定の3倍 米NPO、AIでデータ修正した新研究
2019年10月30日(水)18時05分
11
0
米国の非営利団体クライメト・セントラルは、2050年までに気候変動による沿岸冠水でリスクにさらされる人の数はこれまでに考えられていた規模の3倍以上に達するとみられ、アジア周辺や北米と欧州の複数の都市が海面上昇の影響を受ける可能性がある、との研究結果を発表した。写真は豪雨で冠水したバングラディシュのダッカで2017年7月撮影(2019年 ロイター/Mohammad Ponir Hossain)
米国の非営利団体クライメト・セントラルは29日、2050年までに気候変動による沿岸冠水でリスクにさらされる人の数はこれまでに考えられていた規模の3倍以上に達するとみられ、アジア周辺や北米と欧州の複数の都市が海面上昇の影響を受ける可能性がある、との研究結果を発表した。
同研究は、各国政府が温暖化ガス排出を大幅に抑制できたとしても、水害への予防措置が十分に施されなければ、3億人の人々が住む地域が今世紀半ばまでに少なくとも年に1回は洪水に見舞われるリスクがあると指摘した。
これまでの推定では、そうした被災人口の予想規模は8000万人前後とされていた。リスクにさらされている人口の大半は、中国、バングラデシュ、インド、ベトナムの住民という。
これまでのデータでは、人の住む沿岸地帯の多くがより高い位置にあると算出し、したがって実際より安全であると示唆していた。今回の研究では、人工知能(AI)を駆使してこの算出におけるシステムエラーを修正したという。
同社の代表であるベンジャミン・ストラウス氏は「いまわれわれは、海面上昇と沿岸冠水の脅威がこれまでに考えられていたよりはるかに大きいことを認識した」と述べた。
[ロンドン 29日 ロイター]
https://www.newsweekjapan.jp/stories/world/2019/10/3npoai.php
【気候科学】世界の沿岸部は従来の推定よりも海面上昇に対して脆弱になっている
Nature Communications
2019年10月30日
Climate science: Coasts more vulnerable to sea-level rise than previously thought
Nature Communications
世界の海岸線で、海面上昇と沿岸洪水に対する脆弱性が、従来の推定の3倍に達していることを示唆するモデル研究について報告する論文が掲載される。
今回、Scott KulpとBenjamin Straussは、新しい数値標高モデル(CoastalDEM)を用いて、地球上で極端に高い水位の沿岸海域の影響を受ける人口の評価を行った。CoastalDEMは、従前のモデルの垂直バイアスを低減するために役立つニューラルネットワークを使用しており、全世界で現在の満潮線より低い陸地に合計1億1000万人が居住し、現在の洪水位より低い陸地に2億5000万人が居住していると推定している。この推定結果は、それぞれを2800万人と6500万人とした先行研究による推定人口とは対照的だ。
Kulpたちは、CoastalDEMを用いて、2020年までに温室効果ガス排出量がピークに達する低炭素排出シナリオでは、2100年の時点で予想海面水位より低い陸地に1億9000万人が居住し、温室効果ガスの排出量が21世紀を通じて増加する高排出シナリオでは、2100年の時点で予想洪水位より低い陸地に最大6億3000万人が居住するという見解を示している。現在は、満潮線からの高さが10m未満の陸地に10億人が居住し、洪水位からの高さが1m未満の陸地に2億5000万人が居住しているとKulpたちは推定している。
doi:10.1038/s41467-019-12808-z
「Nature 関連誌注目のハイライト」は、ネイチャー広報部門が報道関係者向けに作成したリリースを翻訳したものです。より正確かつ詳細な情報が必要な場合には、必ず原著論文をご覧ください。
注目のハイライト
10月30日
【気候科学】世界の沿岸部は従来の推定よりも海面上昇に対して脆弱になっているNature Communications
10月30日
【神経科学】アルツハイマー病のアミロイドベータ繊維の構造が明らかにNature Communications
10月30日
【医学研究】肝繊維症の画像化Nature Communications
10月29日
【進化学】鳥の卵の色はなぜ多様なのかNature Ecology & Evolution
10月25日
【気候科学】300年間の薄化で崩壊しやすくなったと考えられる南極の棚氷Scientific Reports
10月24日
【健康】マウスの高塩分摂取が認知機能低下に結び付いているNature
https://www.natureasia.com/ja-jp/research/highlight/13127
• https://www.nature.com/articles/s41467-019-12808-z
• Open Access
• Published: 29 October 2019
New elevation data triple estimates of global vulnerability to sea-level rise and coastal flooding
• Scott A. Kulp &
• Benjamin H. Strauss
Nature Communications volume 10, Article number: 4844 (2019) | Cite this article
Article metrics
• 3899 Altmetric
• Metricsdetails
Abstract
Most estimates of global mean sea-level rise this century fall below 2 m. This quantity is comparable to the positive vertical bias of the principle digital elevation model (DEM) used to assess global and national population exposures to extreme coastal water levels, NASA’s SRTM. CoastalDEM is a new DEM utilizing neural networks to reduce SRTM error. Here we show – employing CoastalDEM—that 190 M people (150–250 M, 90% CI) currently occupy global land below projected high tide lines for 2100 under low carbon emissions, up from 110 M today, for a median increase of 80 M. These figures triple SRTM-based values. Under high emissions, CoastalDEM indicates up to 630 M people live on land below projected annual flood levels for 2100, and up to 340 M for mid-century, versus roughly 250 M at present. We estimate one billion people now occupy land less than 10 m above current high tide lines, including 250 M below 1 m.
Introduction
Driven by climate change, global mean sea level rose 11–16 cm in the twentieth century1,2. Even with sharp, immediate cuts to carbon emissions, it could rise another 0.5 m this century3,4,5,6,7,8,9,10,11,12. Under higher emissions scenarios, twenty-first century rise may approach or in the extremes exceed 2 m in the case of early-onset Antarctic ice sheet instability4,8. Translating sea-level projections into potential exposure of population is critical for coastal planning and for assessing the benefits of climate mitigation, as well as the costs of failure to act.
Land topography and elevation, as represented by DEMs, lie at the foundation of such translation. High-accuracy DEMs derived from airborne lidar are freely available for the coastal United States, much of coastal Australia, and parts of Europe, but are lacking or unavailable in most of the rest of the world. By contrast, SRTM is a near-global satellite-based DEM covering latitudes from 56 south to 60 north and thereby land home to 99.7% of world population (based on 2010 Landscan data13). It is the standard choice for extreme coastal water level (ECWL) exposure analysis covering areas where high-quality elevation data are unavailable or prohibitively expensive14,15,16,17,18,19,20,21.
SRTM models the elevation of upper surfaces and not bare earth terrain. It thus suffers from large error with a positive bias when used to represent terrain elevations. This is especially true in densely vegetated and in densely populated areas22,23,24,25. Mean error in SRTM’s 1–20 m elevation band is 3.7 m in the US and 2.5 m in Australia when using DEMs from airborne lidar as ground truth26. Spaceborne lidar from NASA’s ICESat satellite27, a sparser, noisier and less reliable source of ground truth than airborne lidar, indicates SRTM has a global mean bias of 1.9 m in the same band26. This degree of error leads to large underestimates of ECWL exposure28, and exceeds projected sea-level rise this century under almost any scenario3,4,5,6,7,8,9,10,11,12.
In this article, we present ECWL exposure assessments that address this problem by employing CoastalDEM, a new DEM developed using a neural network to perform nonlinear, nonparametric regression analysis of SRTM error. This model incorporates 23 variables, including population and vegetation indices, and was trained using lidar-derived elevation data in the US as ground truth. CoastalDEM covers the same near-global latitudes as SRTM while reducing vertical bias to decimeter scale (0.01 m and 0.11 m as measured versus airborne lidar in the coastal US and Australia; −0.29 m as tested versus spaceborne lidar globally). CoastalDEM also cuts RMSE roughly in half compared to SRTM26. In low-elevation US coastal areas (where SRTM elevation is less than or equal to 20 m) in which population density exceeds 20,000 per square kilometer, including areas in 14 coastal cities such as Miami, New York City, and Boston, CoastalDEM reduces linear vertical bias from 4.71 m to less than 0.06 m. An overview of the methods used to generate CoastalDEM can be found in the methods section.
Central estimates in the recent literature broadly agree that global mean sea level is likely to rise 20–30 cm by 20503,4,5,6,7,8,9,10. End-of-century projections diverge more, with typical central estimates ranging from 50–70 cm under representative concentration pathway (RCP) 4.5 and 70–100 cm under RCP 8.53,9,10,12, though more recent projections incorporating Antarctic ice sheet dynamics indicate that sea levels may rise 70–100 cm under RCP 4.5 and 100–180 cm under RCP 8.5, and could even exceed 2 m or more in far-tail scenarios4,7,8,11. Via a structured elicitation of opinion, experts now estimate there is a 5 percent chance 21st century sea-level rise will exceed 2 m29. Essentially all estimates are below the vertical bias of SRTM. Of these, we consider two representative sea-level projections for this assessment, labeled here as K143 and K174. K14 is a probabilistic projection that is closely aligned with IPCC findings10,30, while K17 is not probabilistic and emphasizes the possibility of more rapid sea-level rise because of unstable ice-sheet dynamics31. Further details of these models are discussed in the methods section.
Both sets of projections are conditional on global carbon emissions; RCPs 2.6 (low emissions), 4.5 (moderate emissions), and 8.5 (high emissions) are considered for this analysis32. These models use 2000 as the baseline year (zero sea-level rise), which we treat as present-day with respect to sea level for relevant vulnerability estimates. The results we present here are based on median sea-level projections, along with 90% credible intervals when derived from K14, and 90% intervals from simulation frequency distributions when derived from K17 (we abbreviate both interval types as CI).
Because higher and more frequent coastal flooding is a direct impact of sea-level rise33,34, we also assess potential exposure to ECWLs resulting from annual floods added on top of rising seas. We use local one-year return level heights (RL1) from the Global Tides and Surge Reanalysis35. These return levels vary spatially from a 5th percentile of 0.2 m to a 95th percentile of 2.8 m above local mean higher-high water (MHHW)—roughly speaking, the high tide line—across the near-global set of coastal cells assessed in this study (median value, 0.7 m).
We find that assessments using CoastalDEM instead of SRTM multiply median global ECWL exposure by roughly three or more for all scenarios and models considered. The majority of people living on implicated land are in developing countries across Asia, and chronic coastal flooding or permanent inundation threatens areas occupied by more than 10% of the current populations of nations including Bangladesh, Vietnam, and many Small Island Developing States (SIDS) by 2100.
Results
Global
Given each sea level scenario analyzed (Supplementary Table 1), and alternately using SRTM and CoastalDEM, we estimate the number of people on land that may be exposed to coastal inundation—either by permanently falling below MHHW, or temporarily falling below the local annual flood height (Table 1, Supplementary Data 1). Coastal defenses are not considered, but hydrologic connectivity to the ocean is otherwise enforced using connected components analysis. Figure 1 presents permanent inundation surfaces at select locations for median K17/RCP 8.5/2100. Future population growth and migration are also not considered; rather, we use 2010 (essentially current) population density data from Landscan13 to indicate threats relative to present development patterns.
Table 1 Global populations on land at risk
Full size table
Fig. 1
Permanent inundation surfaces predicted by CoastalDEM and SRTM given the median K17/RCP 8.5/2100 sea-level projection. Locations include (a) the Pearl River Delta, China; (b) Bangladesh; (c) Jakarta, Indonesia; and (d) Bangkok, Thailand. Low-lying areas isolated from the ocean are removed from the inundation surface using connected components analysis. Current water bodies are derived from the SRTM Water Body Dataset. Gray areas represent dry land. Axis labels denote latitude and longitude
Full size image
Population exposure to projected sea level or coastal flooding is most commonly expressed as the total estimated exposure below a particular water level (total exposure)14,16,17,19,21,36, but is increasingly also presented as the difference in exposure above a contemporary baseline (marginal exposure)16,21,37. Each approach has complementary strengths and limitations, discussed later. Here, we include marginal exposure values for key findings, while focusing more on total exposure. The latter is simpler and supports a wider and more easily interpretable set of comparisons between CoastalDEM-derived and SRTM-derived results.
For the present day, CoastalDEM estimates a global total of 110 M people on land below the current high tide line and 250 M on land below annual flood levels, in contrast with corresponding SRTM-based estimates of 28 M and 65 M. These values form the basis of the difference between total and marginal exposure estimates.
For one moderate future scenario, sea levels projected by 2050 are high enough to threaten land currently home to a total of 150 (140–170) million people to a future permanently below the high tide line, or a marginal increase of 40 (30–60) million. Total and marginal exposure each rise by another 50 (20–90) million people by end of century. A total of 360 (310–420) million people are on land threatened by annual flood events in 2100, or an extra 110 (60–170) million beyond the contemporary baseline. This case reflects greenhouse gas emissions cuts roughly consistent with warming of 2 °C (emissions scenario RCP 4.5) and assumes a mostly stable Antarctic (sea-level model K14).
In the case of Antarctic instability, a total of 300 (270–340) million people today live on land indicated as vulnerable to an annual flood event by mid-century, rising to as many as 480 (380–630) million by 2100. These values represent marginal increases of 50 (20–90) and 230 (130–380) million from the present, respectively. All 90% CIs given originate from uncertainty in sea-level projections.
More broadly, the effect on estimated ECWL exposure from changing the elevation data used exceeds the combined effects of emissions level, Antarctic behavior, and incorporation of annual flooding, as assessed using SRTM. For example, based on CoastalDEM, the total median current population on land falling below the projected mean higher high water line in 2100 under low emissions and a fairly stable Antarctica (RCP 2.6 and K14) is 190 million. This figure doubles the median SRTM-based estimate of 95 million under high emissions and Antarctic instability (RCP 8.5 and K17), and even exceeds SRTM-based figures under the same scenario after the addition of areas below the annual flood level (170 million).
More straightforwardly, Supplementary Data 2 and 3 tabulate people currently occupying land from 0–10 m MHHW at 1 m intervals, according to CoastalDEM and SRTM, respectively. In previous work using SRTM18, about 640 M people have been estimated to live in the low elevation coastal zone (LECZ), defined as areas below 10 m. Defining the LECZ to reference MHHW instead of EGM96, we find SRTM predicts 780 M people below this threshold, and with CoastalDEM, the estimate rises to just over one billion people. Remarkably, this latter prediction includes 770 M below 5 m, versus 230 M from 5–10 m, illustrating a strong concentration in the lowest areas. The densest 1-m vertical band among the first ten is from 1-to-2 m, with 170 M inhabitants (or 1.7 M per vertical centimeter), pointing to a risky global pattern of development in light of sea-level rise.
National
With both SRTM and CoastalDEM, and regardless of emissions scenario or sea-level model, we find that more than 70% of the total number of people worldwide currently living on implicated land are in eight Asian countries: China, Bangladesh, India, Vietnam, Indonesia, Thailand, the Philippines, and Japan (Fig. 2, Supplementary Data 1). China alone accounts for 15–28% of global ECWL exposure across DEMs, depending upon the scenario, but CoastalDEM increases absolute estimates for China by a factor of roughly three compared to SRTM. Under K14/RCP 4.5, China could see land now home to a total of 43 (29–64) million people below MHHW by end of century, or 57 (30–100) million in the case of Antarctic instability (K17/RCP 4.5). The marginal increases in exposure from baseline are 20 (6–41) million and 34 (7–77 million), respectively. Under the same emissions scenario and either sea-level model, annual flood events at least double the corresponding estimates, threatening land occupied by over 60 million additional people.
Fig. 2
Total populations on vulnerable land. a Current population on land below projected mean higher high water level in 2100 assuming intermediate carbon emissions (RCP 4.5) and relatively stable Antarctic ice sheets (sea level model K14). Estimates based on CoastalDEM. b Factor by which CoastalDEM increases estimates of people on vulnerable land over SRTM in each country under K14/RCP 4.5. Countries wholly north of 60 degrees N are excluded because CoastalDEM is undefined at those latitudes. Source data are provided as a Source Data file. National boundaries based on public domain vector map data by Natural Earth (naturalearthdata.com)
Full size image
In several developing countries south of China, ECWL exposure may be an order of magnitude more serious than previously expected as based on SRTM. As indicated by CoastalDEM, Bangladesh, India, and Vietnam come to rival China in the median number of people living on land implicated by 2100, totaling 21–30 million even under the low emissions scenario (K14/RCP 2.6), compared to 9–19 M today, and with another 10–25 million on land threatened by annual storm surge. Bangladesh, India, Indonesia, and the Philippines see a 5-fold to 10-fold change in estimated current populations below the projected high tide line after applying CoastalDEM. Globally, application of CoastalDEM leads to increased exposure estimates for the great majority of nations (Fig. 3).
Fig. 3
CoastalDEM versus SRTM by country. Each point represents a country, and its position corresponds to estimated total current population on land below the projected mean higher-high water level in 2100 (K14/RCP 4.5) using CoastalDEM (y-axis) versus SRTM (x-axis). The total global value is designated with the red point. Very large differences typically indicate large low-lying areas hydrologically connected to the ocean under CoastalDEM, but not SRTM. Source data are in Supplementary Data 1
Full size image
Percentage rather than absolute exposure serves as a normalized metric of threat (Supplementary Data 4). In Asia, CoastalDEM indicates that even with deep cuts to carbon emissions (K14/RCP 2.6), Bangladesh, Vietnam, and Thailand may, by end-of-century, face high tide lines higher than land now home to 19 (15–25)%, 26 (23–31)%, and 17 (15–18)% of their people, respectively, before accounting for episodic flooding events. These figures correspond to marginal exposure increases of 13 (9–19)%, 5 (2–10)%, and 15 (13–16)% of national populations. Continued high emissions with Antarctic instability (K17/RCP 8.5) could entail land currently home to roughly one-third of Bangladesh’s and Vietnam’s populations permanently falling below the high tide line. It follows that some coastal municipalities within these nations will see even larger proportions of their populations threatened with displacement.
Outside of Asia and excluding the Netherlands, where an extensive flood control network is not captured by any of the elevation models studied, CoastalDEM indicates that 20 other countries are expected to see land currently home to 10% or more of their total populations fall below end-of-century high tide lines (based on median estimates), even under the deep emissions cuts of RCP 2.6. This count is up from two using SRTM. Except for Djibouti, Guyana, and the United Arab Emirates, all of these are island nations, and thirteen are classified by the United Nations as Small Island Developing States (SIDS).
Supplementary Data 1 and 4 provide results for the present, mid-century, and 2100.
Validation
The aspirational outcome of applying CoastalDEM to ECWL exposure analysis is to, as closely as possible, estimate the same amount of coastal vulnerability that a DEM derived from airborne lidar data would. We validate our results by first performing three representative ECWL exposure analyses using lidar-derived data in the US and Australia. In Fig. 4, we plot the relative differences of predicted current population exposure between lidar and each global DEM at different water heights. Values of nearly zero imply a close match between exposure computed using both lidar and the target DEM, while larger absolute values suggest under-estimation or over-estimation of vulnerability. In addition to CoastalDEM and SRTM, we also include the alternative elevation models AW3D30 and MERITDEM, discussed more below.
Fig. 4
The relative difference of computed population ECWL exposure between lidar and four global DEMs. Populations living on land below 1, 2, and 3 m are computed in the US and Australia with each DEM. Zero relative differences indicate both lidar and the given global DEM predict the same number of people below the elevation threshold whereas, for example, −0.5 and 0.5 would indicate that the global DEM underestimation or overestimated by 50%, respectively. Results are given for each US state, as well as at the national scale in the US and Australia. Source data are provided as a Source Data file
Full size image
We find that CoastalDEM strongly and consistently outperforms SRTM (as well as the other global DEMs) with this metric. At 1 m above MHHW, CoastalDEM improves linear relative difference in every state except for New York. Error is reduced from −69% (SRTM) to −43% (CoastalDEM) across the US, and from −77% (SRTM) to −23% (CoastalDEM) in Australia. Even larger improvements are seen at higher water levels, and at 3 m, relative errors in the US and Australia are smaller than −29 and 7%, respectively. We note that while the neural network that generated CoastalDEM was trained on lidar-derived data in the US, Australian lidar data is used only to validate the results, meaning strong results seen here mitigate fears that the model has been overfitted.
Error in the US is dominated by Florida, where an exceptionally large population occupies the coastal plain, and where SRTM vertical error in the southern half of the state is unusually high (exceeding 4 to 10 m). The neural network that generated CoastalDEM did not fully correct this large error. Discounting Florida, US relative error at 1 m drops from −62% (SRTM) to −30% (CoastalDEM)—a comparable improvement to that seen in Australia.
Sensitivity analysis
Spatial autocorrelation commonly characterizes DEM error, including error within SRTM38. SRTM error is strongly correlated with factors such as land slope39, dense vegetation24, and high population density40, which themselves exhibit natural spatial autocorrelation. These features could manifest at any number of spatial scales (some towns may be only a few kilometers wide, while some urban agglomerations and forests are far larger). Furthermore, there exist well-known striping artifacts present in SRTM caused by satellite microadjustments41, resulting, in cases, in multi-meter upward or downward bias across regions that may reach on the order of 100 km wide.
While CoastalDEM makes substantial improvements to SRTM, and includes, in its construction, inputs designed to reduce or eliminate striping, we anticipate that CoastalDEM also suffers from autocorrelated error. We therefore conduct a sensitivity analysis to explore the potential effects of error in CoastalDEM on our population exposure estimates, including the effects of autocorrelated error.
Monte Carlo simulations are regularly used to model DEM error and generate distributions of flood exposure estimates, from which uncertainty may be evaluated38,42,43. Such approaches typically either assume zero spatial autocorrelation, using the DEM’s documented RMSE to generate random error surfaces42,44; or use low-pass filters across the error fields to simulate small-scale autocorrelation45; or employ sequential Gaussian simulations, which require widely dispersed ground-control-point data to accurately measure error statistics across the DEM43,46. The wide range of autocorrelation scale present here makes the second option unsuitable, and with no ground-control-point data available globally, the third is not possible.
Because of our expectations around the importance of spatial autocorrelation, we apply a modified, multi-scale approach to the first of these three methods. Assuming a normal distribution of error centered on zero and using a fixed global standard deviation, we generate 100 error fields using each of 6 different block sizes within which uniform error applies, ranging from 1 pixel (3 arcseconds) to 1 degree. We add the blocked errors to the original CoastalDEM to produce new simulated 3 arcsecond DEMs for computing exposure; the resulting exposure distributions are then evaluated separately for each block resolution. We use CoastalDEM’s RMSE in Australia (2.46 m), as determined using lidar, to serve as the global standard deviation for our error distributions. We choose RMSE from Australia versus the US (RMSE 2.39 m) because the CoastalDEM model was trained in the US (albeit on just a 1% coastal sample). While vertical error will inevitably vary some from place to place, the similarity in error between the US and Australia increases our confidence in the value we employ.
We elect to use a water height of 2 m above MHHW (roughly and generally corresponding to a bad flood in the nearer term or an extreme sea-level scenario for 2100) as a case study. As in the main study, connected components analysis is used to remove isolated areas under the inundation surface before computing exposure. Unmodified CoastalDEM estimates 400 M people worldwide live below this threshold. Table 2 and Supplementary Data 5, respectively, provide global and country-level results for this sensitivity analysis.
Table 2 Global simulated error assessment results
Full size table
Smaller error-block sizes (1-pixel through 1/10-degree resolution, roughly the size of a small city) produce highly consistent exposure estimates at the global scale, though biased low relative to the 400 M predicted without simulated error. This bias may be caused by higher spatial frequency DEM alterations cutting off some low-lying inland areas connected to the ocean through narrow pathways in the original CoastalDEM. Consistent with this mechanism, bias dissipates at larger error-block sizes. Also as autocorrelation scale grows, we see that 90% confidence intervals widen. At the extreme 1 degree resolution, roughly the scale of SRTM striping, the global 90% CI reaches plus or minus 10% about the 400 M median.
Countries also experience widening CI’s across error resolutions, though considerably more rapidly than seen at the global scale. In countries with at least 1 M people below the 2 m threshold, the 90% CI’s are, on average, plus or minus 2% about the median at 1 pixel, 5% at 1 km, 23% at 1/10 degree, 32% at 1/4 degree, 41% at 1/2 degree, and 49% at 1 degree. For example, at the 1-degree-error resolution, Bangladesh, India, and Vietnam have CI’s of (−56 to 54%), (−40 to 27%), and (−34 to 23%) about their respective medians, while China is predictably less sensitive at (−21 to 21%). In general, larger areas of analysis and smaller error blocks lead to less sensitivity in ECWL exposure estimates, because each of these factors leads to larger random samples, making errors more likely to cancel out. Conversely, smaller areas and larger blocks each lead to smaller samples and more sensitivity.
These results suggest that CoastalDEM error exerts little influence on our global estimates, but reasonable caution should be applied when interpreting national scale assessments, particularly for smaller countries such as the SIDS. That said, we note that the 1-degree simulations represent worst-case scenarios, because they assume that CoastalDEM’s RMSE derives exclusively from the largest considered spatial scale. Given the known factors at many spatial scales that contribute to DEM error, this assumption is unrealistic. Assessing characteristic error autocorrelation scales is beyond the scope of this study, but realistic CIs will be considerably narrower than implied by the 1-degree scale.
Discussion
Despite improvements, elevation dataset error remains an important limitation in this study. We see that CoastalDEM still underestimates population exposure in both the US and Australia when compared to lidar-derived DEMs, suggesting the current assessment does not fully eliminate the bias in exposure estimates based on SRTM. CoastalDEM may still experience difficulty in dense cities, where exceptionally tall buildings in even the lowest-lying areas can cause SRTM elevations erroneously above 20 m. Since CoastalDEM is defined only where SRTM elevation is lower than or equal to 20 m, such areas are disregarded in this analysis, leading to some underestimation of exposure.
Older global scale DEMs, such as GLOBE47 and GTOP03048, have been used in previous work, and generally predict higher coastal flood exposure than SRTM19,20. However, their extremely high vertical errors (up to 100 m RMSE in both cases), low horizontal resolution (1 km), and spatial inconsistency in quality make them unreliable for ECWL vulnerability assessments. Their use for research has faded in comparison with SRTM, given its higher horizontal resolution and order-of-magnitude lower error. More recently, other DEM’s have been released, such as AW3D3049 and MERITDEM50. AW3D30 is a digital surface model primarily derived from stereo optical satellite imagery, and does not specifically attempt to improve vertical bias in either urban or forested areas. MERITDEM, like CoastalDEM, is based off of SRTM. It uses regression analysis to remove vertical error correlated with a number of vegetation metrics. However, MERITDEM does not seek to correct errors due to urban development. For sake of comparison, the analyses described in this article were repeated for these DEMs, and included in Supplementary Data 1 and 4. Results from both AW3D30 and MERITDEM, including US/Australia ECWL exposure error (Fig. 4), are generally consistent with those derived from SRTM, and so we maintain that these DEMs are equally inadequate for assessing coastal vulnerability.
Future modeling efforts may improve estimation of terrain elevations in tall-building districts and areas affected by SRTM striping. Ultimately, the most accurate assessments of vulnerability to rising seas, especially for smaller areas, will require development and public release of improved coastal area elevation datasets building directly off of new high resolution observations increasingly collected by satellites today.
Another limitation of this assessment comes from the population dataset Landscan, which is a 1 km2 resolution model of ambient population density. While Landscan is widely used in the research literature, it cannot capture any bias toward or away from development within the lowest-lying coastal areas at sub-kilometer spatial scales. GRUMP is another population dataset with the same horizontal resolution, though it involves less sophisticated spatial modeling and is available only through 2000. It models nighttime (rather than ambient) population density51, and has been shown to produce notably higher predictions of exposure to ECWL20. Gridded Population of the World52 is another alternative, based directly on census data without further modeling. While nominally at 1 km horizontal resolution, the data is piecewise constant between administrative boundaries, meaning its effective resolution is actually much coarser than Landscan. Newer datasets, such as Worldpop53 and the High Resolution Settlement Layer54, are anticipated to model population densities with higher accuracy at finer resolution, but are not yet available globally.
We emphasize that this analysis combines future water level projections with contemporary population densities. Results should therefore not be taken as projected impacts. Rather, they reflect the portion of presently developed land at risk in the future, which we interpret as a threat indicator. Efforts to integrate projected population growth, migration, economic development and coastal defenses into ECWL exposure projections have begun19,36,55. However, the spatial scales of socioeconomic projections remain very coarse compared to the scales at which elevation and current development data are available, posing a stiff challenge to their meaningful integration into analyses where fine-scale detail is critical. In addition, behavioral and economic responses to rising seas are likely to be unpredictable, due to the largely unprecedented nature and scale of the problem.
The vulnerability model employed in this analysis, a bathtub model where we classify all land below a given water height and hydrologically connected to the ocean as exposed to extreme coastal water levels, presents another partial limitation of the study. While this approach is reasonable in indicating land threatened with permanent inundation due to higher sea levels, it tends to overestimate exposure from episodic flooding, especially at small spatial scales56,57. It is likely that hydrodynamic models would predict less vulnerability to one-year floods than we estimate here. Areas accordingly misclassified as exposed to annual flooding would nonetheless likely face relatively frequent inundation risks.
Furthermore, this analysis assumes a static coastal topography, with the exception of a linear model of vertical land motion implicit in the sea-level projections used. Erosion, wetland migration/accretion, and other morphological processes are not considered. It is difficult to predict how these factors affect the uncertainty of our results, especially since sea-level change may trigger complex process responses. However, we note that armored, developed, and maintained shorelines in urban areas, where vulnerable populations are concentrated, may generally be less susceptible to such factors than undeveloped land.
This study focuses on estimating populations occupying land below future high tide lines or annual flood levels, but results also indicate that some 110 M people live below MHHW today (with many more below annual flood lines). Several explanations are possible. First, elevation error may drive the finding. However, in the US and Australia, CoastalDEM identifies fewer people living below MHHW (0.9 M and 69,000, respectively) than lidar-based analysis does (1.7 M and 75,000), consistent with our more general finding that CoastalDEM tends to underestimate coastal exposure relative to lidar.
Second, other sources of error may be important, including from the population data used and from the sea level data and tidal models used to determine local MHHW. A more detailed lidar-based analysis employing high resolution (block-level) US Census data58 and NOAA’s nearly continuous model for local MHHW59 within the US cuts the original lidar-based estimate of 1.7 M nearly in half, to 0.9 M residents on land below MHHW. If these US results are indicative, and global population and MHHW estimates inflate exposure values derived from lidar elevation data, they likely also inflate values derived from CoastalDEM. Higher accuracy and higher resolution population, sea level and tidal inputs are likely important for improving coastal exposure assessments in the future.
Third, many people today do in fact live on land below (or just above) MHHW, behind the protection of levees or other defenses. In the US, these account for 0.8 M out of the 0.9 M residents that our more detailed lidar analysis identifies as today occupying land below MHHW. Globally at present, levees and seawalls protect low-lying populations in many major deltas, such as around Shanghai, the Netherlands and New Orleans, and in areas experiencing rapid subsidence, such as parts of Jakarta and Tokyo. However, levee location data are not globally available, to our knowledge, and so are not incorporated into this analysis.
Fourth and finally, many people today do live in unprotected areas subject to frequent coastal flooding (if not below the high tide line), such as in Bangladesh, or in boats or structures on or above the water (such as homes on stilts). These possibilities are likely to be most common in developing countries, and to be poorly documented.
The levees, seawalls and other defenses and accommodations currently protecting tens or hundreds of millions of coastal-area residents globally point to the potential for protecting ever-larger areas as seas rise. At the same time, current coastal defenses should not be assumed adequate to protect against future sea levels and storms without continued maintenance and, likely, enhancement. These countervailing possibilities point to the merits of reporting results based both on total ECWL exposure and on marginal increases in exposure from the contemporary baseline. Total exposure recognizes the potential vulnerability of all populations on low-lying coastal lands as sea levels rise. Marginal exposure highlights new populations of concern, while leaving out populations in areas that may be defended at present, and thus may be more likely to be defended in the future.
Even in light of the limitations identified, this research, using a significantly improved model of coastal elevations, provides new best estimates of the vulnerability of populated low-lying areas to rising oceans at global and national scales. Reliability increases with the size of the area evaluated, and with the water level considered; thus, global assessments for end-of-century sea levels and floods, under high sea-level scenarios, should be considered most robust. Analysis reveals a developed global coastline three times more exposed to extreme coastal water levels than previously thought. Even with low carbon emissions and stable Antarctic ice sheets, leading to optimistically low future sea levels, we find that the global impacts of sea-level rise and coastal flooding this century will likely be far greater than indicated by the most pessimistic past analyses relying on SRTM. These results point to great need for the development and public release of improved terrain elevation datasets for coastal areas, for example via the high-resolution imagery and lidar point clouds increasingly collected by satellite today. There is also great need for improved population data; data on the location, height and condition of coastal-area levees and seawalls; and improved global sea-level and tidal models.
If our findings stand, coastal communities worldwide must prepare themselves for much more difficult futures than may be currently anticipated. Recent work has suggested that, even in the US, sea-level rise this century may induce large-scale migration away from unprotected coastlines, redistributing population density across the country and putting great pressure on inland areas60. It is difficult to extrapolate such projections and their impacts to more resource-constrained developing nations, though historically, large-scale migration events have posed serious challenges to political stability, driving conflict61. Further research on global-scale modeling of the timing, locations, and intensity of migratory responses to increased coastal flooding is urgently needed to minimize the potential human harm caused by such threats.
Methods
Sea-level projections
We use two sea-level models for this assessment. K143 employs a probabilistic approach and includes very little contribution from Antarctica in its central projections. K174 links physical models of ice sheet loss to the projection framework established in K14, thus emphasizing the possibility of early-onset Antarctic instability31. However, the ice sheet model parameters used were not derived from probability distributions. Unlike K14, the resulting projection distributions produced by K17 are therefore considered simulation frequency distributions, rather than probabilistic ones. While more recent work62 suggests that these Antarctic projections may be biased high, the resulting overall sea-level projections align roughly with the high end of what the sea level research community broadly expects29. Both models incorporate spatially explicit submodels for all climatic components of sea-level rise considered. They each also incorporate nonclimatic background contributions, such as glacial isostatic adjustment and sediment compaction. Leveraging sea-level records collected at 1022 PSMSL tide gauges worldwide, both K14 (updated in 20174) and K17 employ a Gaussian process model to estimate nonclimatic contributions at points on a 2° × 2° grid along the entire global coastline. Results for both models at the tide gauge and grid point locations are included in Supplementary Datasets 3 and 4 of Kopp et al.4.
CoastalDEM
A multilayer perceptron (MLP) artificial neural network, a computational model often used for highly nonlinear non-parametric regression, was employed to predict the vertical error present at any SRTM pixel sample. MLP’s are made up of layers of nodes in a weighted, directed graph, starting with an input layer (in our case, a 23-dimensional vector of known attributes at the target location) and ending with an output layer (1-dimensional error prediction at the target location). The neural network is trained by using lidar-derived elevation data in the US63 as ground truth, iteratively adjusting weights in the graph to most accurately reproduce desired targets given the training set of samples. Our training set was made of over 51 million samples, and the 23 variables included neighborhood elevation values, land slope, population density, vegetation density, canopy height, and local SRTM deviations from ICESat altitude observations64. After training, the MLP predicted and removed SRTM errors at every pixel in the DEM with elevation between 1 and 20 m (inclusive). Details on the implementation and vertical error assessment of CoastalDEM were published earlier26. For this report, we used median resampling to convert CoastalDEM to a 3-arcsecond horizontal resolution.
Vertical datum conversion
We convert all elevation data to a common vertical reference frame (datum) for valid intercomparisons, electing the tidal datum mean higher high water (MHHW). MHHW is roughly equivalent to local high tide line and captures spatial variation in both mean sea level (MSL) and tidal amplitude. We use the globally extensive MSL model MSS_CNES_CLS_1565, based on a 1993–2012 record of satellite sea surface height measurements from TOPEX/Poseidon, and referenced to the GLAS ellipsoid at 1-arcminute horizontal resolution. We also employ MHHW deviations from MSL provided by Mark Merrifield, University of Hawaii, developed using the model TPX0866 at 2-arcminute horizontal resolution. Using NOAA’s VDatum tool59 version 3.7, we convert CoastalDEM, SRTM, AW3D30 and MERITDEM, plus the GLAS-referenced MHHW elevations, to a common ellipsoidal datum (WGS84). This allows us to subtract the elevation map of MHHW from each DEM to produce our final elevation maps above local MHHW.
A similar approach is taken in converting 1-year return levels to MHHW. The Global Tides and Surge Reanalysis, as distributed, is referenced to local MSL, so we use the MHHW-MSL deviation surfaces to change its vertical datum to MHHW.
Exposure analysis
Employing a modified bathtub model, we threshold each pixel in the DEMs to produce inundation surfaces at 0–10 m above MHHW. These inundation surfaces are computed at 1 m intervals with SRTM and AW3D30 (equivalent to their vertical resolutions), and at 0.25 m intervals with CoastalDEM and MERITDEM (which have continuous vertical resolutions). The surfaces are then refined using connected components analysis to remove all low-lying sub-threshold areas that the analysis indicates to be isolated by topography from the ocean.
To assess population exposure, we employ the LandScan 2010 High Resolution global Population Data Set, which estimates total populations living in 1 km2 cells13. We refine this data using the SRTM Water Body Data Set, which defines land cells at up to 1-arcsecond resolution (30 m). We resample Landscan to align our DEM grids, assuming zero population in water cells, while proportionally increasing the population density in land cells to ensure total population in each original 1 km square remains unchanged.
The population density grids are integrated under each 0.25 m-interval inundation surface, and tabulated according to the smallest administrative boundaries defined by the Global Administrative Areas (GADM) 2.0 Data Set67. In general, these administrative units are roughly the size of US counties, or smaller. The local sea-level rise projections and 1-year return level heights, now referenced to local MHHW, are then computed and added at the centroid of each boundary by linearly interpolating from nearby sample points from the corresponding models. At the scale of these administrative units, the sea-level rise and RL1 gradients are relatively small, so any local factors affecting water heights are captured. Populations on land under each of these water heights are then estimated using linear interpolation between the 0.25 m interval analyses. Results are aggregated to larger administrative areas, such as states and nations, as needed.
Reporting summary
Further information on research design is available in the Nature Research Reporting Summary linked to this article.
Code availability
The methods described in this article were implemented using custom Matlab (R2017b), Python, and C++ code. Due to licensing restrictions by Climate Central, this code is not publicly available.
Data availability
All exposure analyses (national populations on vulnerable land) that support the findings of our study are available within this article and its supplementary information files. The datasets SRTM, AW3D30, MERITDEM, Landscan 2010, and GADM are publicly available from their respective owners. The 3-arcsecond (90-m) version of CoastalDEM used in this analysis is available at no cost from Climate Central for non-commercial research use.
References
1. 1.
Hay, C. C., Morrow, E., Kopp, R. E. & Mitrovica, J. X. Probabilistic reanalysis of twentieth-century sea-level rise. Nature 517, 481–484 (2015).
o ADS
o CAS
o Article
o Google Scholar
2. 2.
Dangendorf, S. et al. Reassessment of 20th century global mean sea level rise. Proc. Natl Acad. Sci. 114, 5946–5951 (2017).
o ADS
o CAS
o Article
o Google Scholar
3. 3.
Kopp, R. E. et al. Probabilistic 21st and 22nd century sea-level projections at a global network of tide-gauge sites. Earth’s. Future 2, 383–406 (2014).
o ADS
o Article
o Google Scholar
4. 4.
Kopp, R. E. et al. Evolving Understanding of Antarctic Ice-Sheet Physics and Ambiguity in Probabilistic Sea-Level Projections. Earth’s. Future 5, 1217–1233 (2017).
o ADS
o Article
o Google Scholar
5. 5.
Nauels, A., Meinshausen, M., Mengel, M., Lorbacher, K. & Wigley, T. M. L. Synthesizing long-term sea level rise projections the MAGICC sea level model v2.0. Geoscientific Model Dev. 10, 2495–2524 (2017).
o ADS
o Article
o Google Scholar
6. 6.
Nauels, A., Rogelj, J., Schleussner, C.-F., Meinshausen, M. & Mengel, M. Linking sea level rise and socioeconomic indicators under the Shared Socioeconomic Pathways. Environ. Res. Lett. 12, 114002 (2017).
7. 7.
Bakker, A. M. R., Wong, T. E., Ruckert, K. L. & Keller, K. Sea-level projections representing the deeply uncertain contribution of the West Antarctic ice sheet. Sci. Rep. 7, 3880 (2017).
8. 8.
Wong, T. E., Bakker, A. M. & Keller, K. Impacts of Antarctic fast dynamics on sea-level projections and coastal flood defense. Clim. Change 144, 347–364 (2017).
o ADS
o CAS
o Article
o Google Scholar
9. 9.
Jevrejeva, S., Moore, J. & Grinsted, A. Sea level projections to AD2500 with a new generation of climate change scenarios. Glob. Planet. Change 80, 14–20 (2012).
10. 10.
Stocker, T. et al. Climate Change 2013: The Physical Science basis. Contribution of Working Group I to the Fifth Assessment Report of the Intergovernmental Panel on Climate Change (Cambridge University Press, Cambridge, New York, 2013).
11. 11.
Le Bars, D., Drijfhout, S. & de Vries, H. A high-end sea level rise probabilistic projection including rapid Antarctic ice sheet mass loss. Environ. Res. Lett. 12, 044013 (2017).
12. 12.
Jackson, L. P. & Jevrejeva, S. A probabilistic approach to 21st century regional sea-level projections using RCP and High-end scenarios. Glob. Planet. Change 146, 179–189 (2016).
o ADS
o Article
o Google Scholar
13. 13.
Bright, E. A., Coleman, P. R., Rose, A. N. & Urban, M. L. Landscan 2010. https://landscan.ornl.gov (2011).
14. 14.
Hallegatte, S., Green, C., Nicholls, R. J. & Corfee-Morlot, J. Future flood losses in major coastal cities. Nat. Clim. Change 3, 802–806 (2013).
o ADS
o Article
o Google Scholar
15. 15.
Neumann, T. & Ahrendt, K. Comparing the “Bathtub Method” with MIKE 21 HD Flow Model for Modelling Storm Surge Indundation: Case Study Kiel Fjord. In: RADOST Report Series (Rostock, Germany, 2013).
16. 16.
Neumann, B., Vafeidis, A. T., Zimmermann, J. & Nicholls, R. J. Future Coastal Population Growth and Exposure to Sea-Level Rise and Coastal Flooding—A Global Assessment. PLoS ONE 10, e0118571 (2015).
17. 17.
Rasmussen, D. J. et al. Extreme sea level implications of 1.5 °C, 2.0 °C, and 2.5 °C temperature stabilization targets in the 21st and 22nd centuries. Environ. Res. Lett. 13, 034040 (2018).
o ADS
o Article
o Google Scholar
18. 18.
McGranahan, G., Balk, D. & Anderson, B. The rising tide: assessing the risks of climate change and human settlements in low elevation coastal zones. Environ. Urban 19, 17–37 (2007).
o Article
o Google Scholar
19. 19.
Hinkel, J. et al. Coastal flood damage and adaptation costs under 21st century sea-level rise. Proc. Natl Acad. Sci. USA 111, 3292–3297 (2014).
o ADS
o CAS
o Article
o Google Scholar
20. 20.
Lichter, M., Vafeidis, A. T., Nicholls, R. J. & Kaiser, G. Exploring data-related uncertainties in analyses of land area and population in the “Low-Elevation Coastal Zone” (LECZ). J. Coast. Res. 274, 757–768 (2011).
o Google Scholar
21. 21.
Jongman, B., Ward, P. J. & Aerts, J. C. Global exposure to river and coastal flooding: long term trends and changes. Glob. Environ. Change 22, 823–835 (2012).
o Article
o Google Scholar
22. 22.
Tighe, M. & Chamberlain, D. Accuracy Comparison of the SRTM, ASTER, NED, NEXTMAP USA Digital Terrain Model over Several USA Study Sites DEMs. In: Proceedings of the ASPRS/MAPPS 2009 Fall Conference (2009).
23. 23.
LaLonde, T., Shortridge, A. & Messina, J. The Influence of Land Cover on Shuttle Radar Topography Mission (SRTM) Elevations in Lowrelief Areas. Trans. GIS 14, 461–479 (2010).
o Article
o Google Scholar
24. 24.
Shortridge, A. & Messina, J. Spatial structure and landscape associations of SRTM error. Remote Sens. Environ. 115, 1576–1587 (2011).
o ADS
o Article
o Google Scholar
25. 25.
Becek, K. Assessing Global Digital Elevation Models Using the Runway Method: The Advanced Spaceborne Thermal Emission and Reflection Radiometer Versus the Shuttle Radar Topography Mission Case. IEEE Trans. Geosci. Remote Sens. 52, 4823–4831 (2014).
o ADS
o Article
o Google Scholar
26. 26.
Kulp, S. A. & Strauss, B. H. CoastalDEM: a global coastal digital elevation model improved from SRTM using a neural network. Remote Sens. Environ. 206, 231–239 (2018).
o ADS
o Article
o Google Scholar
27. 27.
Zwally, H. et al. ICESat’s laser measurements of polar ice, atmosphere, ocean, and land. J. Geodynamics 34, 405–445 (2002).
o ADS
o Article
o Google Scholar
28. 28.
Kulp, S. & Strauss, B. H. Global DEM Errors Underpredict Coastal Vulnerability to Sea Level Rise and Flooding. Front. Earth Sci. 4, 36 (2016).
29. 29.
Bamber, J. L., Oppenheimer, M., Kopp, R. E., Aspinall, W. P. & Cooke, R. M. Ice sheet contributions to future sea-level rise from structured expert judgment. Proc. Natl Acad. Sci. USA 116, 11195–11200 (2019).
o ADS
o CAS
o Article
o Google Scholar
30. 30.
Church, J. A. et al. Sea-level rise by 2100. Science 342, 1445 (2013).
o ADS
o CAS
o Article
o Google Scholar
31. 31.
DeConto, R. M. & Pollard, D. Contribution of Antarctica to past and future sea-level rise. Nature 531, 591–597 (2016).
o ADS
o CAS
o Article
o Google Scholar
32. 32.
IIASA. RCP Database 2.0.5, https://tntcat.iiasa.ac.at/RcpDb (2009).
33. 33.
Tebaldi, C., Strauss, B. H. & Zervas, C. E. Modelling sea level rise impacts on storm surges along US coasts. Environ. Res. Lett. 7, 014032 (2012).
34. 34.
Buchanan, M. K., Kopp, R. E., Oppenheimer, M. & Tebaldi, C. Allowances for evolving coastal flood risk under uncertain local sea-level rise. Clim. Change 137, 347–362 (2016).
o ADS
o Article
o Google Scholar
35. 35.
Muis, S. et al. A global reanalysis of storm surges and extreme sea levels. Nat. Commun. 7, 11969 (2016).
36. 36.
Hauer, M. E., Evans, J. M. & Mishra, D. R. Millions projected to be at risk from sea-level rise in the continental United States. Nat. Clim. Change 6, 691–695 (2016).
o ADS
o Article
o Google Scholar
37. 37.
Hallegatte, S. et al. Assessing climate change impacts, sea level rise and storm surge risk in port cities: a case study on Copenhagen. Clim. Change 104, 113–137 (2010).
o Article
o Google Scholar
38. 38.
Gesch, D. B. Best Practices for Elevation-Based Assessments of Sea-Level Rise and Coastal Flooding Exposure. Front. Earth Sci. 6 (2018).
39. 39.
Ludwig, R. & Schneider, P. Validation of digital elevation models from SRTM X-SAR for applications in hydrologic modeling. ISPRS J. Photogramm. Remote Sens. 60, 339–358 (2006).
o ADS
o Article
o Google Scholar
40. 40.
Gamba, P., Acqua, F. D. & Houshmand, B. SRTM data characterization in urban areas. nternational Archives of Photogrammetry Remote Sensing and Spatial. Inf. Sci. 34, 55–58 (2002).
o Google Scholar
41. 41.
Rodriguez, E. et al. An assessment of the SRTM topographic products, Technical Report JPL D-31639 (2005).
42. 42.
Wechsler, S. P. & Kroll, C. N. Quantifying DEM Uncertainty and its Effect on Topographic Parameters. Photogrammetric Eng. Remote Sens. 72, 1081–1090 (2006).
o Article
o Google Scholar
43. 43.
Wechsler, S. P. Uncertainties associated with digital elevation models for hydrologic applications: a review. Hydrol. Earth Syst. Sci. 11, 1481–1500 (2007).
o ADS
o Article
o Google Scholar
44. 44.
Cooper, H., Fletcher, C., Chen, Q. & Barbee, M. Sea-level rise vulnerability mapping for adaptation decisions using LiDAR DEMs. Prog. Phys. Geogr. 37, 745–766 (2013).
o Article
o Google Scholar
45. 45.
Enwright, N. et al. The Impact of Lidar Elevation Uncertainty on Mapping Intertidal Habitats on Barrier Islands. Remote Sens. 10, 5 (2017).
o ADS
o Article
o Google Scholar
46. 46.
Leon, J. X., Heuvelink, G. B. M. & Phinn, S. R. Incorporating DEM Uncertainty in Coastal Inundation Mapping. PLoS ONE 9, e108727 (2014).
47. 47.
Hastings, D. A. et al. Global Land One-kilometer Base Elevation (GLOBE) Digital Elevation Model, Documentation, Volume 1.0, http://www.ngdc.noaa.gov/mgg/topo/report/globedocumentationmanual.pdf (1999).
48. 48.
USGS. GTOPO30 Documentation, https://webgis.wr.usgs.gov/globalgis/gtopo30/gtopo30.htm#h31 (1999).
49. 49.
Tadono, T. et al. Generation of the 30 M-Mesh Global Digital Surface Model by Alos Prism. ISPRS—Int. Arch. Photogramm., Remote Sens. Spat. Inf. Sci. XLI-B4, 157–162 (2016).
o Article
o Google Scholar
50. 50.
Yamazaki, D. et al. A high-accuracy map of global terrain elevations. Geophys. Res. Lett. 44, 5844–5853 (2017).
o ADS
o Article
o Google Scholar
51. 51.
Balk, D., Francesca, P., Yetman, G., Deichmann, U. & Nelson, A. The distribution of people and the dimension of place: methodologies to improve the global estimation of urban extents. In Proceedings of the Urban Remote Sensing Conference (2005).
52. 52.
Doxsey-Whitfield, E. et al. Taking Advantage of the Improved Availability of Census Data: A First Look at the Gridded Population of the World, Version 4. Pap. Appl. Geogr. 1, 226–234 (2015).
o Article
o Google Scholar
53. 53.
Tatem, A. J. WorldPop, open data for spatial demography. Sci. Data 4 170004 (2017).
54. 54.
CIESIN. High Resolution Settlement Layer (HRSL), https://www.ciesin.columbia.edu/data/hrsl/ (2016).
55. 55.
Desmet, K. et al. Evaluating the Economic Cost of Coastal Flooding. Techical Report. National Bureau of Economic Research, Cambridge, http://www.nber.org/papers/w24918.pdf (2018).
56. 56.
Seenath, A., Wilson, M. & Miller, K. Hydrodynamic versus GIS modelling for coastal flood vulnerability assessment: Which is better for guiding coastal management? Ocean Coast. Manag. 120, 99–109 (2016).
o Article
o Google Scholar
57. 57.
Vafeidis, A. T. et al. Water-level attenuation in global-scale assessments of exposure to coastal flooding: a sensitivity analysis. Nat. Hazards Earth Syst. Sci. 19, 973–984 (2019).
o ADS
o Article
o Google Scholar
58. 58.
US Census Bureau. TIGER/Line Shapefiles Technical Documentation. Techical Report. https://www.census.gov/geo/maps-data/data/pdfs/tiger/tgrshp2012/TGRSHP2012_TechDoc.pdf (2012).
59. 59.
Parker, B., Hess, K., Milbert, D. & Gill, S. A national vertical datum transformation tool. Sea Technol. 44, 10–16 (2003).
o Google Scholar
60. 60.
Hauer, M. E. Migration induced by sea-level rise could reshape the US population landscape. Nat. Clim. Change 7, 321–325 (2017).
o ADS
o Article
o Google Scholar
61. 61.
Reuveny, R. Climate change-induced migration and violent conflict. Polit. Geogr. 26, 656–673 (2007).
o Article
o Google Scholar
62. 62.
Edwards, T. L. et al. Revisiting Antarctic ice loss due to marine ice-cliff instability. Nature 566, 58–64 (2019).
o ADS
o CAS
o Article
o Google Scholar
63. 63.
NOAA. Digital Coast Coastal Lidar, http://coast.noaa.gov/digitalcoast/data/coastallidar (2015).
64. 64.
Schutz, B. E., Zwally, H. J., Shuman, C. A., Hancock, D. & DiMarzio, J. P. Overview of the ICESat Mission. Geophys. Res. Lett. 32, L21S01 (2005).
65. 65.
Aviso. MSS CNES-CLS15, https://www.aviso.altimetry.fr/en/data/products/auxiliary-products/mss.html (2015).
66. 66.
Egbert, G. D. & Erofeeva, S. Y. Efficient inverse modeling of barotropic ocean tides. J. Atmos. Ocean. Technol. 19, 183–204 (2002).
o ADS
o Article
o Google Scholar
67. 67.
University of Berkeley, Museum of Vertebrate Zoology and International Rice Research Institute. Global Administrative Areas (Boundaries), http://www.gadm.org/, https://library.mcmaster.ca/maps/geospatial/global-administrative-areas-gadm (2012).
Download references
Acknowledgements
The authors gratefully acknowledge Maya Buchanan, Michael Oppenheimer and Claudia Tebaldi for their thoughtful insights and comments on the manuscript. This research was supported by the Schmidt Family Foundation, the V. Kann Rasmussen Foundation, as well as One Earth, a project of Sustainable Markets Foundation, in partnership with the Leonardo DiCaprio Foundation, and grants from the National Science Foundation (ICER-1663807) and the National Aeronautics and Space Administration (80NSSC17K0698).
Author information
Affiliations
1. Climate Central, Palmer Square #402, Princeton, NJ, 08542, USA
o Scott A. Kulp
o & Benjamin H. Strauss
Contributions
S.K. and B.S. conceived and designed the analysis. S.K. performed the analysis. S.K. and B.S. wrote the paper.
Corresponding author
Correspondence to Scott A. Kulp.
Ethics declarations
Competing interests
The authors declare no competing interests.
Additional information
Peer review information Nature Communications thanks the anonymous reviewer(s) for their contribution to the peer review of this work. Peer reviewer reports are available.
Publisher’s note Springer Nature remains neutral with regard to jurisdictional claims in published maps and institutional affiliations.
Supplementary information
Peer Review File
Supplementary Information
Description of Additional Supplementary Files
Supplementary Data 1
Supplementary Data 2
Supplementary Data 3
Supplementary Data 4
Supplementary Data 5
Reporting Summary
Source data
Source Data
Rights and permissions
Open Access This article is licensed under a Creative Commons Attribution 4.0 International License, which permits use, sharing, adaptation, distribution and reproduction in any medium or format, as long as you give appropriate credit to the original author(s) and the source, provide a link to the Creative Commons license, and indicate if changes were made. The images or other third party material in this article are included in the article’s Creative Commons license, unless indicated otherwise in a credit line to the material. If material is not included in the article’s Creative Commons license and your intended use is not permitted by statutory regulation or exceeds the permitted use, you will need to obtain permission directly from the copyright holder. To view a copy of this license, visit http://creativecommons.org/licenses/by/4.0/.
投稿コメント全ログ コメント即時配信 スレ建て依頼 削除コメント確認方法

すべてのページの引用、転載、リンクを許可します。確認メールは不要です。引用元リンクを表示してください。